In today’s digital world, data is one of the most valuable resources. With businesses collecting enormous volumes of information every second, analyzing this data efficiently has become crucial. This is where Big Data Analytics comes into play. It involves using advanced techniques to extract insights, uncover patterns, and make data-driven decisions from vast datasets.
But what exactly is Big Data Analytics, how does it work, and why is it so important? In this article, we will explore how Big Data Analytics works, its types, benefits, examples, and more.
What is Big Data Analytics?
Big Data Analytics refers to the process of analyzing massive and complex datasets that are too large for traditional data processing tools. These datasets may include both structured data (such as organized financial records) and unstructured data (such as customer reviews or social media posts). By applying advanced analytical techniques, businesses can discover hidden patterns, trends, correlations, and insights from the data.
Big Data Analytics is not limited to any single industry. Its applications range from healthcare and retail to finance, manufacturing, transportation, and more. For example, Amazon uses Big Data to recommend products, while Visa applies it to detect fraudulent transactions.
How Does Big Data Analytics Work?
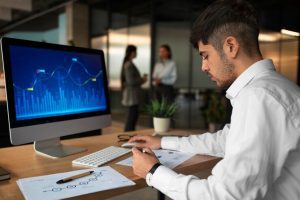
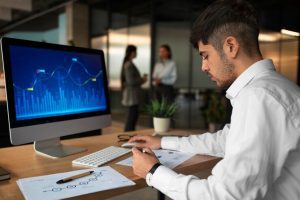
Big Data Analytics is a multi-step process. It begins with collecting data and ends with actionable insights. Here’s a detailed breakdown of each step:
Data Collection:
Data is gathered from various sources such as social media, website traffic, sensors, customer reviews, and sales data. In industries like healthcare, data can come from patient records, while in retail, data can come from point-of-sale systems.
Data Cleaning (Preprocessing):
Once collected, the data must be cleaned. This involves fixing errors, removing duplicates, and addressing missing or incorrect data. Think of this step as sifting through a pile of rubble to find valuable pieces of information. For example, inaccurate sensor data in transportation systems could lead to incorrect predictions, so this stage is crucial.
Data Processing:
After cleaning, the data is processed into a format suitable for analysis. This might involve structuring the data and ensuring it is organized for use with analytical tools. Think of this step like preparing ingredients before cooking a meal.
Data Analysis:
This is the core stage where statistical methods, machine learning models, and mathematical algorithms are used to uncover trends and patterns. This is where businesses get valuable insights, such as predicting customer preferences or identifying market trends.
Data Visualization:
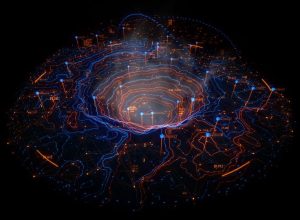
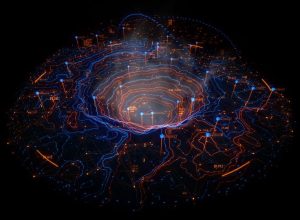
The results from the analysis are often presented visually through charts, graphs, and dashboards to make complex data more understandable. For example, Tableau is a popular tool that transforms data into interactive visuals for decision-makers.
Data Storage and Management:
The analyzed data is then stored and managed efficiently for future use. Data protection and regulatory compliance are vital in this stage, especially in industries like finance where security is paramount.
Continuous Learning and Improvement:
Big Data Analytics is an ongoing process. Companies constantly collect and analyze data to uncover new insights and make better decisions.
Types of Big Data Analytics
Big Data Analytics isn’t a one-size-fits-all solution. There are different types, each suited for specific purposes:
Descriptive Analytics:
This type focuses on understanding past events. For example, a social media platform might use descriptive analytics to measure user engagement, such as the number of likes or shares a post receives.
Diagnostic Analytics:
This digs deeper into the reasons behind past events. In healthcare, diagnostic analytics can identify causes of high patient re-admissions by analyzing patient history.
Predictive Analytics:
As the name suggests, this type predicts future outcomes based on past data. For instance, weather forecasting uses predictive analytics to predict future weather conditions by analyzing historical patterns.
Prescriptive Analytics:
Prescriptive analytics not only predicts outcomes but also recommends actions. In e-commerce, it can recommend the best pricing strategy to maximize profits based on customer behavior.
Real-time Analytics:
Real-time analytics processes data as it is generated. In the finance industry, real-time analytics helps traders make decisions based on live market data.
Spatial Analytics:
This type focuses on location-based data. In urban management, spatial analytics helps optimize traffic flow by analyzing data from sensors and cameras.
Text Analytics:
Text analytics analyzes unstructured data like customer reviews or social media posts. Hotels use this to improve guest satisfaction by analyzing feedback.
Tools Used in Big Data Analytics
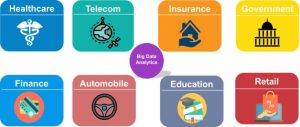
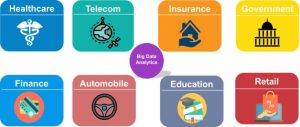
Several tools make Big Data Analytics more manageable. Here are some commonly used ones:
Hadoop:
Think of Hadoop as a giant warehouse for storing and processing massive datasets. Amazon uses Hadoop to store user data and provide personalized recommendations.
Spark:
Spark is a fast data processing engine. Netflix uses Spark to quickly analyze user viewing habits and recommend new shows.
NoSQL Databases:
Databases like MongoDB provide flexible storage solutions, helping companies like Airbnb store user booking data efficiently.
Tableau:
This visualization tool turns data into interactive visuals, making it easier for decision-makers to understand trends.
Python and R:
These programming languages are widely used for data analysis. Kaggle competitions often feature these tools for predictive modeling.
Machine Learning Frameworks (TensorFlow):
Used by companies like Airbnb, machine learning frameworks help predict outcomes, such as which properties are most likely to be booked.
Benefits of Big Data Analytics
Big Data Analytics offers numerous advantages to businesses across industries:
Informed Decisions:
Companies like Walmart use Big Data to optimize stock levels, reducing waste and ensuring they have the right products in stock.
Enhanced Customer Experience:
Amazon‘s personalized product recommendations, driven by Big Data, provide a tailored shopping experience for each customer.
Fraud Detection:
In the finance industry, companies like Visa rely on Big Data to detect and prevent fraudulent transactions, safeguarding customer data.
Optimized Logistics:
FedEx uses Big Data to optimize delivery routes, cutting down delivery times and reducing environmental impact.
Improved Healthcare:
Big Data helps in precise diagnoses and disease prediction. Hospitals use analytics to reduce patient re-admissions and improve care quality.
Cost Reduction:
By optimizing operations and reducing inefficiencies, Big Data can help companies save money. For example, Delta Airlines uses analytics to optimize flight schedules.
Examples of Big Data Analytics in Action
- Amazon: Big Data powers Amazon’s recommendation system, suggesting products based on past purchases and browsing history.
- MasterCard: Big Data helps MasterCard detect fraudulent transactions in real time, providing an extra layer of security for customers.
- Netflix: Netflix uses Big Data to analyze viewer preferences and recommend shows, improving the user experience.
- General Electric (GE): In the manufacturing sector, GE uses Big Data to predict when machinery needs maintenance, reducing downtime and improving productivity.
Challenges of Big Data Analytics
While the benefits are vast, Big Data Analytics comes with its own set of challenges:
- Data Overload:
Managing massive datasets can be overwhelming. For instance, Twitter generates over 6,000 tweets per second, making it challenging to sift through relevant data. - Data Quality:
Inaccurate or incomplete data can lead to flawed analysis. For example, incorrect sensor readings in weather forecasting could result in incorrect predictions. - Privacy Concerns:
With so much personal data involved, companies must navigate the fine line between providing personalized experiences and infringing on privacy. - Security Risks:
Data security is a major concern, especially in industries like banking, where sensitive financial information must be protected from cyberattacks. - Costs:
Implementing and maintaining Big Data Analytics systems can be expensive. Businesses must ensure that the benefits outweigh the costs.
Conclusion
Big Data Analytics is transforming the way businesses operate, enabling them to make smarter, data-driven decisions. From improving customer experiences to optimizing logistics and predicting future trends, Big Data Analytics offers numerous benefits across industries. However, challenges such as managing data overload, ensuring privacy, and controlling costs must be addressed.
In today’s data-driven world, Big Data Analytics is not just a tool for large corporations—it’s a game-changer for businesses of all sizes. As more companies adopt these practices, the future of Big Data Analytics looks promising, with endless opportunities to gain insights and drive success.