Meet Dr. Emily Alonso
Dr. Emily Alonso is a renowned expert in artificial intelligence with over 15 years of experience in machine learning research and development. She’s passionate about building transparent and reliable AI systems and has published extensively on the topic of AI explainability and debugging. In this article, Dr. Alonso dives into the world of AI debugging, offering practical strategies for data scientists and machine learning developers.
Headings:
- The Challenge of Black Box AI
- Why Debugging AI Matters
- Unveiling the Secrets: Techniques for AI Debugging
- 3.1. Visualization Techniques
- 3.2. Feature Importance Analysis
- 3.3. Counterfactual Explanations
- Case Study: Debugging a Loan Approval Model
- Best Practices for Explainable AI
- The Future of AI Debugging
Unveiling the Black Box: Techniques for AI Debugging
The power of artificial intelligence (AI) is undeniable, revolutionizing numerous industries. However, complex AI models often operate like black boxes, their decision-making processes shrouded in mystery. This lack of transparency can pose significant challenges, making it difficult to identify and fix errors, ensure fairness, and build trust in AI systems.
Why Debugging AI Matters
Effective debugging is paramount for ensuring the success of AI projects. Here’s why:
- Improved Accuracy: Debugging helps identify and rectify biases and errors that can lead to inaccurate predictions.
- Enhanced Explainability: By understanding how AI models arrive at decisions, we can explain them to stakeholders and build trust.
- Fairness and Bias Detection: Debugging techniques can help uncover hidden biases within AI models, promoting fairness and ethical AI development.
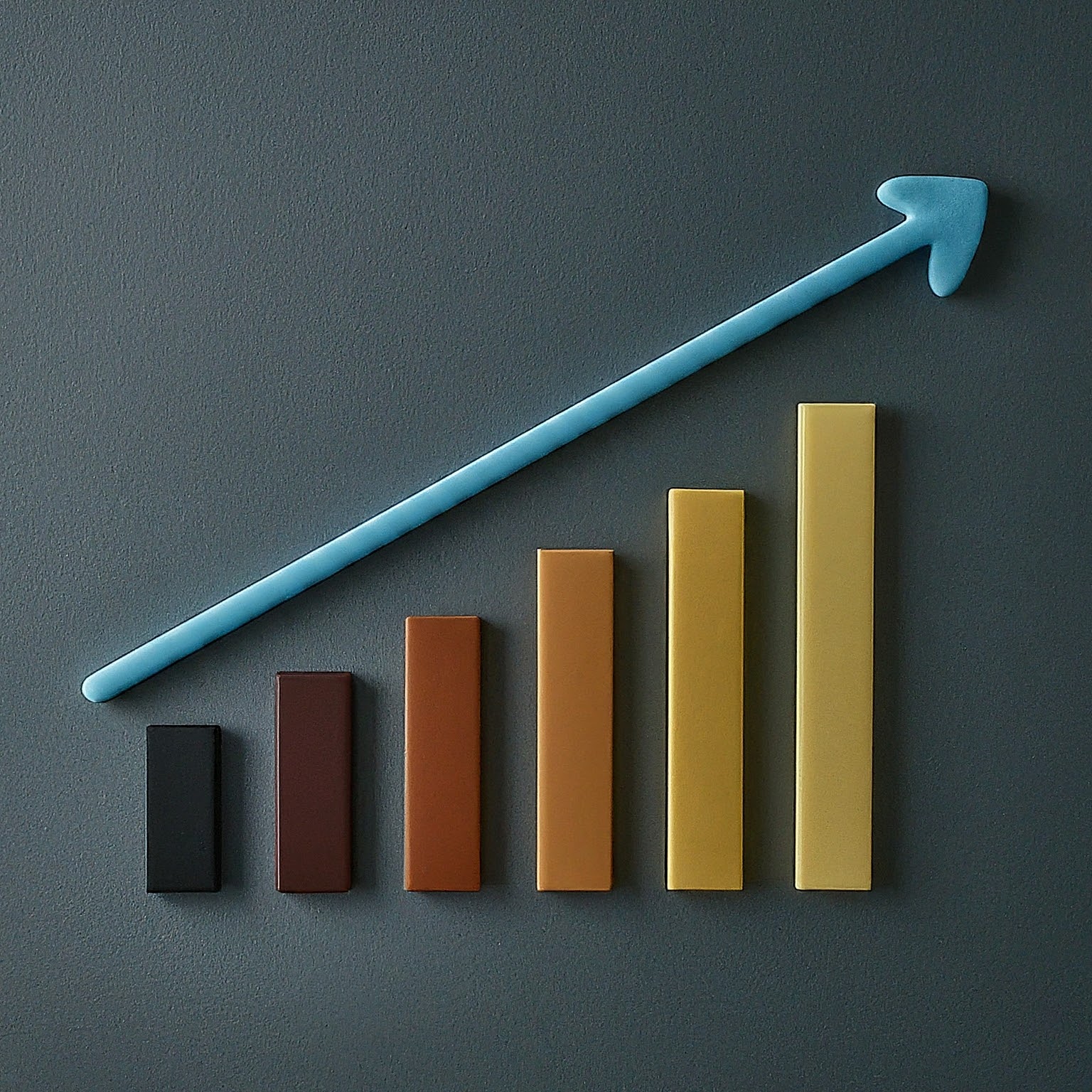
Unveiling the Secrets: Techniques for AI Debugging
Several techniques can be employed to shed light on the inner workings of complex AI models:
3.1. Visualization Techniques
- Saliency Maps: These visual representations highlight the input features that most influence the model’s output, allowing developers to pinpoint crucial factors for decision-making.
- Decision Trees: Visualizing the decision-making process within tree-based models can aid in understanding the logic behind each prediction.
3.2. Feature Importance Analysis
This technique quantifies the importance of each input feature in influencing the model’s output. Features with low importance might be irrelevant or redundant, leading to potential errors.
3.3. Counterfactual Explanations
This method explores alternative scenarios (“what-if” analysis) to explain a particular prediction. This can help users understand how changing specific inputs might affect the model’s output.
Case Study: Debugging a Loan Approval Model
Imagine a loan approval model consistently rejects loan applications from a specific demographic group. Debugging techniques can help identify potential biases within the model. By analyzing feature importance and utilizing counterfactual explanations, developers can pinpoint the cause of bias and rectify the model to ensure fair loan approval practices.
Best Practices for Explainable AI (XAI)
- Choose interpretable models: When possible, opt for AI models known for their inherent explainability, such as decision trees or rule-based systems.
- Collect diverse data: Training AI models on diverse and representative datasets helps mitigate bias and improve generalizability.
- Integrate explainability tools: Utilize frameworks and libraries specifically designed to enhance the explainability of AI models during development.
The Future of AI Debugging
As AI continues to evolve, so too will the field of AI debugging. We can expect advancements in explainable AI techniques, automated debugging tools, and standardized methodologies for ensuring transparency and reliability in complex AI systems.
By embracing these techniques and best practices, data scientists and machine learning developers can unlock the full potential of AI, fostering trust, fairness, and continuous improvement in the ever-evolving world of artificial intelligence.
Table 1: Common AI Debugging Techniques
Technique | Description | Benefits |
---|---|---|
Visualization Techniques (Saliency Maps, Decision Trees) | Visually represent the model’s decision-making process | Improves understanding of model behavior and identifies influential features. |
Feature Importance Analysis | Quantifies the importance of each input feature | Helps identify irrelevant or redundant features that might impact model accuracy. |
Counterfactual Explanations | Explores alternative scenarios to explain a specific prediction | Provides insights into how changing inputs might affect model output. |
Note: This article is not an exhaustive list of AI debugging techniques. New methods and tools are constantly emerging in this rapidly developing field.