The Role of Machine Learning in Predicting Market Trends
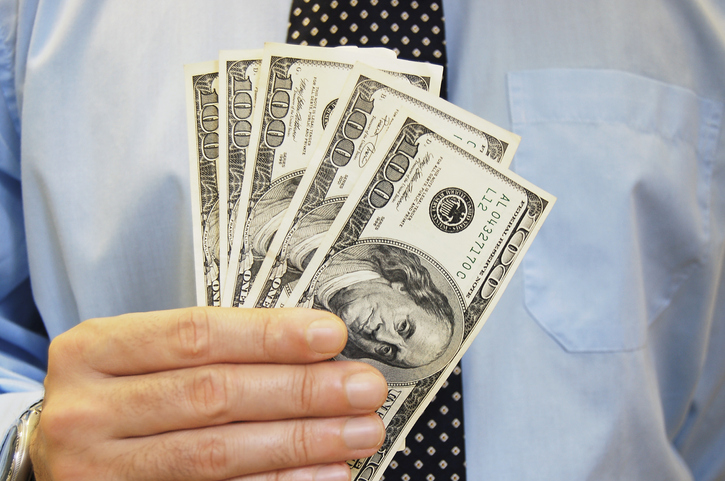
In recent years, machine learning has transformed various industries by offering data-driven insights and facilitating more effective decision-making processes. One area where (ML) has shown particular promise is in predicting market trends. As markets become increasingly volatile and complex, traditional forecasting methods are often limited in their ability to process vast datasets and deliver timely predictions. This article explores the role of in predicting market trends, detailing its advantages, challenges, applications, and future prospects.
Understanding Machine Learning in Market Predictions
is a branch of artificial intelligence (AI) that enables systems to learn from data and improve their performance over time without explicit programming. In market trend predictions, ML algorithms process large datasets, analyze historical patterns, and predict future movements based on identified trends. learning’s capacity to handle unstructured data, recognize complex patterns, and adapt to new information makes it an invaluable tool for market analysis and forecasting.
Key Aspects of Machine Learning in Market Predictions
- Data Processing: ML models can handle both structured and unstructured data, analyzing historical and real-time data to forecast trends.
- Pattern Recognition: By identifying underlying patterns, machine models can predict market behavior based on past trends.
- Adaptability: Machine learning systems continuously learn and refine their algorithms, enhancing the accuracy of predictions over time.
Applications of Machine Learning in Predicting Market Trends
Machine learning has diverse applications in predicting market trends, with uses across stock markets, real estate, consumer markets, and economic forecasting.
- Stock Market Prediction: ML algorithms are widely used in stock market forecasting. Models such as neural networks and reinforcement learning analyze price movements, historical data, and news sentiment to forecast stock prices.
- Real Estate Market Forecasting: Machine learning can analyze variables like location, property prices, interest rates, and economic conditions to help predict property price trends.
- Consumer Behavior Analysis: Machine learning models process data from social media, online behavior, and purchase history to anticipate shifts in consumer preferences and demands.
- Economic Indicators Prediction: Governments and financial institutions use to analyze macroeconomic indicators like inflation rates, employment statistics, and GDP growth, which helps them foresee economic shifts and prepare accordingly.
Advantages of Machine Learning in Market Predictions
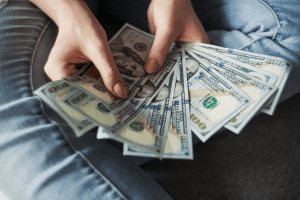
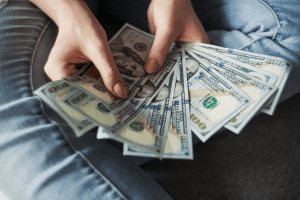
Machine learning has brought about several significant improvements to market forecasting processes. Here are some key advantages:
- Accuracy and Precision: Machine learning can process large volumes of data, resulting in more accurate predictions by capturing subtle trends that human analysts might overlook.
- Speed and Efficiency: ML algorithms can analyze massive datasets and deliver predictions in real-time, making it easier for analysts to respond quickly to market changes.
- Automation: By automating data analysis, reduces human error and enhances efficiency in market predictions.
- Predictive Analytics: ML-powered predictive analytics allow businesses to make informed decisions by understanding potential outcomes based on current data.
Challenges in Using Machine Learning for Market Predictions
Despite its many benefits, the integration of machine learning into market trend prediction comes with its own set of challenges:
- Data Quality: Machine learning models rely heavily on high-quality data. Inaccurate or incomplete data can lead to flawed predictions.
- Model Interpretability: Complex ML models like deep learning are often seen as “black boxes,” making it difficult to interpret their predictions.
- Market Volatility: Unpredictable events like natural disasters or economic crises can significantly impact market trends, limiting the accuracy of ML predictions.
- Overfitting: Some machine learning models may overfit historical data, leading to inaccurate predictions if future market behavior deviates significantly.
Comparative Analysis of Traditional Forecasting vs. Machine Learning in Market Trends
Aspect | Traditional Forecasting | Machine Learning Forecasting |
---|---|---|
Data Processing | Limited to structured data | Can handle both structured and unstructured data |
Speed and Efficiency | Slower, often requires human input | Faster, can process data automatically |
Accuracy | Moderate, depends on analyst expertise | Higher, based on complex algorithms and data patterns |
Adaptability | Limited adaptability | Continuously learns and adapts to new data |
Interpretability | Easier to interpret | Often complex and challenging to interpret |
Real-Time Analysis | Limited | Real-time analysis possible |
Machine Learning Models Used in Market Trend Predictions
Various machine learning models have proven effective in predicting market trends. Here’s a breakdown of some commonly used algorithms:
Machine Learning Model | Application in Market Trends | Strengths | Limitations |
---|---|---|---|
Linear Regression | Forecasting continuous price movements | Simple, interpretable | May underperform in complex markets |
Decision Trees | Segmenting market data into distinct patterns | Handles both categorical and numerical data | Can overfit without pruning |
Neural Networks | Predicting stock prices, identifying patterns | Powerful pattern recognition | Requires large datasets and processing |
Support Vector Machines (SVM) | Classifying market trends, anomaly detection | Effective with complex patterns | Can be computationally intensive |
Random Forests | Portfolio management, real estate forecasting | Reduces overfitting, robust results | Slower for large datasets |
Reinforcement Learning | Dynamic trading strategies, portfolio optimization | Learns from actions and outcomes | Complex setup, requires extensive data |
Future Prospects of Machine Learning in Market Trend Predictions
Machine learning’s role in predicting market trends is anticipated to grow as technology advances. The integration of AI with is expected to provide even greater predictive capabilities, enabling businesses to analyze global events, social media sentiment, and macroeconomic data in real time. Enhanced computational power, cloud computing, and the development of more sophisticated algorithms are likely to improve model accuracy and enable even broader applications across financial and economic sectors.
Potential Areas of Future Growth
- Augmented Machine Learning: Combining human expertise with to improve interpretability and decision-making accuracy.
- Increased Use of Deep Learning Models: Deep learning models will be increasingly capable of identifying highly complex market patterns.
- AI-Powered Real-Time Predictions: With advancements in processing speed, models will enable quicker and more responsive forecasting for volatile markets.
Emerging Technologies Enhancing Machine Learning for Market Predictions
As the capabilities of machine learning evolve, emerging technologies like natural language processing (NLP) and big data analytics are enhancing ML’s effectiveness in market predictions. NLP enables models to interpret sentiment from news articles, social media, and other unstructured data sources, providing insights into public opinion and potential market impacts. Big data analytics, on the other hand, allows machine models to handle vast amounts of information from diverse sources, from economic indicators to global events, creating a more comprehensive view of the market landscape. Together, these technologies empower models to offer predictions that reflect both quantitative data and real-world sentiment, helping businesses stay ahead of trends.
Ethical and Privacy Considerations in Machine Learning Market Predictions
While machine learning offers exciting opportunities for market prediction, it also raises ethical and privacy concerns. Algorithms used to predict trends often rely on extensive data collection, which can include personal information from individuals across digital platforms. This data collection process necessitates strict privacy measures to protect user information and maintain transparency about how data is used. Additionally, reliance on for market predictions can sometimes lead to unintended consequences, such as exacerbating market volatility or creating biases in investment strategies. Addressing these ethical considerations is crucial to ensuring that applications are fair, secure, and respectful of individual privacy while driving progress in market trend prediction.
Introduction to Machine Learning in Market Prediction
Machine learning (ML) has rapidly become a key technology in predicting market trends, transforming how analysts, investors, and businesses approach market forecasting. Traditional market prediction models often struggle to account for the complexity and volatility of modern financial markets. Machine learning offers a solution by enabling systems to learn from vast datasets, recognize patterns, and make predictions based on historical and real-time data. This approach can improve the accuracy of market forecasts, providing a more dynamic and data-driven understanding of future market movements.
Data Processing and Pattern Recognition
One of the core strengths of machine learning lies in its ability to process and analyze large volumes of data, including structured data like stock prices and trading volumes, as well as unstructured data such as news articles, social media posts, and financial reports. By utilizing advanced algorithms, ML models can detect patterns and correlations that are often too complex for traditional analysis. For example, sentiment analysis can be performed on social media or news sources to gauge public perception and predict potential market reactions to specific events, such as earnings reports, geopolitical developments, or product launches.
Adapting to Market Changes with Deep Learning
Machine learning models, particularly deep learning algorithms, are highly adaptive to changes in market conditions. Deep learning networks, such as neural networks, can analyze historical data and identify long-term trends, but they can also adjust their predictions based on new data, improving over time. This adaptability is crucial in markets where conditions can shift rapidly. By continuously learning from fresh data, ML models can stay relevant and maintain accuracy in their predictions, even during periods of high volatility or market disruptions.
Algorithmic Trading and Real-Time Decision Making
A major application of machine learning in market prediction is algorithmic trading, where trading strategies are based on predictions generated by ML models. These algorithms can process large quantities of market data in real time, making instantaneous decisions and executing trades far quicker than human traders. By capitalizing on minor fluctuations in market prices or other inefficiencies, machine learning-driven trading systems can optimize returns and reduce risks. This has revolutionized the way institutional investors and hedge funds operate, providing them with a competitive edge in fast-paced financial markets.
Challenges and Limitations of Machine Learning in Market Prediction
Despite its numerous advantages, the use of machine learning in predicting market trends is not without its challenges. One key concern is overfitting, where a model becomes overly specialized to historical data and fails to generalize to new market conditions. Additionally, ML models are highly dependent on the quality and diversity of the data they are trained on. Biased or incomplete datasets can lead to inaccurate predictions. Furthermore, the unpredictability of external events—such as political crises, natural disasters, or sudden changes in investor sentiment—can make market predictions difficult, as these factors are hard to quantify and may not be fully captured in the data. Therefore, while machine learning enhances market forecasting, it is not a foolproof method and should be used in conjunction with human expertise and other analytical tools.
Conclusion
Machine learning has revolutionized the way we approach market predictions, offering businesses a faster, more accurate, and adaptable way to anticipate market changes. From stock prices to real estate trends and consumer behaviors, enables powerful predictive insights that can transform strategic decision-making. Although challenges remain, particularly concerning data quality, interpretability, and market volatility, machine role in market trend forecasting is poised to grow, leading to smarter, data-driven insights for businesses worldwide.