In today’s digital age, the amount of data generated every second is staggering. With the advent of big data, organizations have access to unprecedented volumes of information. Predictive analytics, a branch of advanced analytics, leverages this data to make predictions about future trends, behaviors, and outcomes. By analyzing current and historical data, predictive analytics allows businesses to make more informed decisions, improve customer experiences, and increase efficiency.
This article explores how big data fuels predictive analytics, delves into its benefits, and presents real-world case studies that illustrate its transformative power.
What is Big Data?
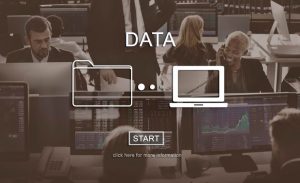
Big data refers to massive volumes of data—both structured and unstructured—that traditional databases and processing software can’t handle effectively. With sources such as social media, online transactions, IoT devices, and customer interactions, data is constantly being generated at high velocity, with vast variety, and in immense volumes (often called the “three Vs” of big data).
Harnessing big data requires specialized tools and techniques to store, analyze, and visualize it, and one of the most impactful applications of big data is predictive analytics.
Understanding Predictive Analytics
Predictive analytics uses statistical algorithms, machine learning, and data mining techniques to identify patterns and forecast future events based on historical data. By analyzing trends and extracting insights, predictive analytics helps businesses answer “what could happen?” rather than “what has happened?”
This forward-looking approach is invaluable for decision-making across various industries, from healthcare and finance to marketing and logistics.
The Benefits of Big Data in Predictive Analytics
1. Enhanced Decision-Making
Big data and predictive analytics allow companies to go beyond intuition and make data-driven decisions. By analyzing past data trends, predictive analytics offers insights into future outcomes, helping leaders anticipate challenges and take proactive measures.
2. Improved Customer Experience
Understanding customer preferences and behavior is critical to creating a satisfying user experience. By leveraging big data, predictive analytics can reveal insights into customer sentiment, buying patterns, and potential churn rates, enabling businesses to tailor their offerings and communication.
3. Cost Optimization
By predicting demand and understanding customer needs, companies can optimize inventory management, reduce waste, and cut costs. Predictive maintenance, for example, can anticipate machinery breakdowns, helping companies to schedule timely repairs and avoid expensive downtime.
4. Risk Mitigation
In sectors like finance and insurance, predictive analytics plays a pivotal role in risk management. Analyzing large datasets of historical transactions and customer profiles allows for better assessment of credit risk, fraud detection, and even market fluctuations, which is crucial for informed investment decisions.
5. Increased Efficiency and Productivity
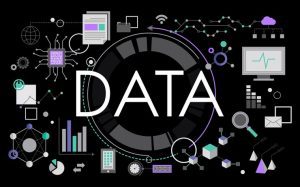
Organizations can increase operational efficiency by identifying patterns in workflow data, production rates, and employee performance metrics. Predictive analytics enables businesses to streamline processes and allocate resources more effectively.
Real-World Case Studies: Big Data and Predictive Analytics in Action
1. Healthcare: Predicting Patient Outcomes and Optimizing Treatment Plans
In healthcare, big data and predictive analytics have opened doors to personalized treatment plans and improved patient outcomes. For instance, researchers at Mount Sinai Hospital developed a predictive analytics platform that analyzes patient data from electronic health records (EHRs) to identify patients at risk of chronic conditions, such as diabetes and heart disease. By leveraging big data, they can intervene early, thus reducing hospital readmission rates and improving patient care.
Additionally, predictive analytics has been instrumental in managing the COVID-19 pandemic. By analyzing data on virus spread, healthcare providers and governments could forecast infection rates, allocate resources, and create contingency plans more effectively.
2. Retail: Enhancing Customer Experience and Sales Forecasting
Retail giants like Amazon and Walmart rely on predictive analytics to forecast demand, manage inventory, and improve customer experiences. Walmart uses predictive analytics to analyze purchasing patterns and anticipate inventory needs during holidays or special events. By analyzing big data on customer preferences, product popularity, and seasonal trends, Walmart ensures that stores are adequately stocked, thereby enhancing customer satisfaction and maximizing sales.
Amazon, on the other hand, uses predictive analytics for personalized recommendations. By analyzing a customer’s browsing history, past purchases, and demographic data, Amazon’s algorithms predict products a customer is likely to buy, thereby increasing engagement and driving sales.
3. Finance: Fraud Detection and Credit Risk Assessment
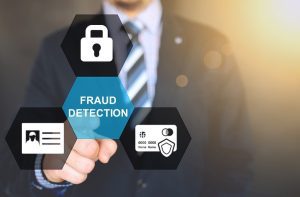
Banks and financial institutions were among the first adopters of predictive analytics. They use big data to identify fraudulent transactions by analyzing patterns in transaction data, flagging anomalies that deviate from typical behavior. For instance, JP Morgan Chase developed an AI-driven model that analyzes millions of transactions daily to identify fraudulent activities, protecting customers from fraud and reducing financial losses.
Predictive analytics is also used in credit risk assessment. By analyzing big data on a customer’s financial history, credit score, and spending patterns, banks can determine the likelihood of a loan being repaid. This helps in creating tailored loan packages and managing risk effectively.
4. Manufacturing: Predictive Maintenance and Quality Control
Manufacturing industries use big data and predictive analytics to enhance operational efficiency and reduce maintenance costs. For example, General Electric (GE) leverages predictive maintenance to monitor the health of their jet engines and predict potential malfunctions before they occur. By analyzing data from sensors embedded in machinery, predictive models can forecast equipment failure, allowing for timely repairs and reducing downtime.
Moreover, predictive analytics assists in quality control by analyzing data from production lines. Patterns of defects or anomalies can be identified, enabling manufacturers to address issues proactively and maintain high product quality.
Key Tools and Technologies for Big Data Predictive Analytics
Apache Hadoop:
Hadoop’s distributed storage and processing capabilities make it a popular choice for handling big data. By distributing data across multiple servers, Hadoop enables efficient data processing, essential for large-scale predictive analytics.
Apache Spark:
Spark offers in-memory data processing, allowing for faster analysis. Its machine learning library, MLlib, supports predictive modeling and is widely used in big data analytics.
Tableau:
As a data visualization tool, Tableau helps organizations turn big data into actionable insights. By creating interactive dashboards, it allows for easier interpretation of predictive analytics results.
Python and R:
These programming languages are popular for data science and predictive modeling due to their extensive libraries and community support.
TensorFlow and PyTorch:
For deep learning-based predictive analytics, TensorFlow and PyTorch are widely used, especially in image recognition, language processing, and other advanced predictive tasks.
Challenges of Implementing Big Data in Predictive Analytics
1. Data Privacy and Security
With increasing data regulations like GDPR, ensuring customer data privacy is a top priority. Predictive analytics requires access to vast amounts of data, raising concerns about data security and ethical data usage.
2. Data Quality and Integration
Data from different sources may vary in quality and format, making it challenging to integrate and analyze effectively. Organizations need to invest in data cleansing and normalization to ensure accurate predictive insights.
3. High Implementation Costs
Building a predictive analytics infrastructure requires significant investment in technology and talent. Organizations must consider the long-term benefits and ROI of such investments.
4. Interpretability of Results
Predictive analytics often involves complex machine learning algorithms, making it difficult for non-technical stakeholders to interpret results. Explainable AI (XAI) and user-friendly dashboards can help bridge this gap.
Future Trends in Big Data and Predictive Analytics
As technology advances, the future of predictive analytics promises to be even more powerful and pervasive. Here are some trends to watch:
AI-Powered Predictive Analytics:
Artificial intelligence, particularly deep learning, is set to enhance the predictive power of analytics. AI can identify intricate patterns in big data that traditional models may miss, leading to more accurate forecasts.
Edge Computing:
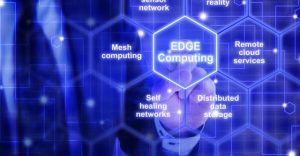
With the rise of IoT devices, data can now be processed closer to its source, reducing latency and enhancing predictive capabilities. Edge computing enables real-time predictive analytics, which is crucial for industries like autonomous vehicles and healthcare.
Enhanced Data Privacy Technologies:
Privacy-preserving techniques, such as federated learning, allow predictive models to learn from big data without accessing sensitive information directly, addressing data privacy concerns.
Predictive Analytics in the Cloud:
Cloud platforms like AWS, Google Cloud, and Microsoft Azure provide scalable infrastructure for big data analytics, allowing organizations to leverage predictive analytics without heavy upfront investments.
Conclusion
Big data has revolutionized the field of predictive analytics, enabling organizations to make proactive decisions based on insights drawn from vast datasets. From healthcare and finance to retail and manufacturing, predictive analytics offers significant benefits, helping organizations optimize operations, enhance customer experiences, and manage risk effectively. However, the journey isn’t without challenges, as data privacy, quality, and interpretability continue to be critical concerns.
As technology continues to evolve, the combination of big data and predictive analytics will only become more integral to decision-making processes. Organizations that leverage this dynamic duo effectively will be well-positioned to thrive in the data-driven future.