Climate change poses a global challenge that demands innovative and scalable solutions. Artificial Intelligence (AI) is emerging as a key player in addressing these challenges. From improving energy efficiency to enhancing renewable energy systems and monitoring environmental changes, AI is driving a technological revolution to combat climate change. This article explores the benefits, real-world examples, and case studies to demonstrate how AI is transforming the fight against climate change.
1. Benefits of AI in Climate Change Solutions
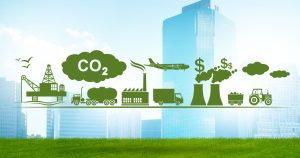
A. Optimizing Energy Efficiency
AI-driven systems are revolutionizing how we use energy in residential, commercial, and industrial spaces. By analyzing patterns in energy consumption, AI can predict energy needs and optimize HVAC (heating, ventilation, and air conditioning) systems for maximum efficiency.
- Benefit: Reduced energy costs and significant reductions in greenhouse gas emissions.
B. Enhancing Climate Predictions
Accurate climate modeling and weather forecasting are crucial for disaster preparedness. AI leverages vast datasets to predict extreme weather events with high accuracy, enabling governments and communities to take timely action.
- Benefit: Lives and resources saved through better planning and mitigation strategies.
C. Monitoring Environmental Changes
From deforestation to wildlife tracking, AI-powered tools can analyze satellite imagery and sensor data to detect changes in the environment in real-time. This helps policymakers and conservationists take swift action.
- Benefit: Enhanced conservation efforts and informed policy-making.
D. Boosting Renewable Energy Efficiency
AI plays a critical role in optimizing renewable energy systems like wind and solar. It predicts energy production and improves grid management, ensuring efficient use of resources.
- Benefit: Lower costs and improved reliability of renewable energy systems.
E. Reducing Industrial Emissions
Industries are major contributors to global carbon emissions. AI helps analyze production processes to identify inefficiencies and reduce emissions.
- Benefit: More sustainable and eco-friendly manufacturing practices.
2. Real-World Examples of AI in Climate Change Solutions
A. BrainBox AI and Energy Efficiency
BrainBox AI’s ARIA platform uses AI to optimize HVAC systems in buildings. Deployed in over 14,000 buildings across 20+ countries, the platform has reduced energy costs by 25% and decreased emissions significantly.
B. Google’s Climate Prediction Models
Google uses AI to improve weather forecasting and climate models. By analyzing complex climate patterns, Google AI helps communities prepare for extreme weather events.
C. AI for Wildlife Conservation
Organizations like the World Resources Institute use AI to analyze satellite data and track deforestation. Tools like Global Forest Watch help identify illegal logging activities and protect forest ecosystems.
D. Renewable Energy Optimization by DeepMind
DeepMind, an AI subsidiary of Google, uses machine learning to predict wind energy output 36 hours in advance. This optimization has increased the value of wind energy by 20%.
E. Sustainable Manufacturing with Siemens
Siemens uses AI to monitor industrial emissions and optimize production lines. This has helped industries significantly reduce their carbon footprint.
3. Case Studies
BrainBox AI Reduces Energy Emissions
BrainBox AI implemented its ARIA platform in a commercial building in Canada. Within six months, the building reduced its energy consumption by 25% and cut CO2 emissions by over 50 metric tons.
- Impact: Demonstrates how scalable AI solutions can make buildings more sustainable.
AI and Deforestation Monitoring
Global Forest Watch used AI to monitor illegal deforestation in the Amazon rainforest. By analyzing satellite images, the AI identified hotspots of illegal activity, prompting government intervention.
- Impact: Protected thousands of hectares of rainforest and curbed illegal logging.
Wind Energy Optimization in the US
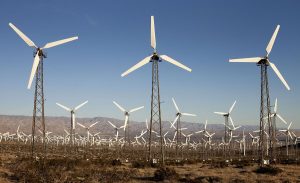
DeepMind’s AI optimized wind energy production in the Midwest US. The model’s ability to predict energy output 36 hours in advance helped improve grid reliability and increase renewable energy use.
- Impact: Boosted renewable energy adoption and reduced dependency on fossil fuels.
AI in Flood Prediction
The Indian state of Assam implemented AI-based flood forecasting models developed by Google. These models predicted floods with over 90% accuracy, allowing communities to evacuate in time.
- Impact: Saved hundreds of lives and minimized property damage.
4. Challenges in Using AI for Climate Solutions (Enhanced)
While AI offers groundbreaking solutions, there are several challenges to its widespread implementation in addressing climate change. Understanding these challenges is crucial to developing strategies that minimize their impact.
A. High Energy Consumption of AI Systems
AI models, particularly those that rely on deep learning, require significant computational power. Training these models involves processing large datasets, which demands high energy usage. Data centers, which house servers and computational infrastructure, are a major source of this energy consumption.
- Example: According to a report by OpenAI, training a large-scale AI model can emit as much carbon as five cars over their lifetime.
- Potential Solutions: Developing energy-efficient AI algorithms and using renewable energy to power data centers can reduce AI’s environmental footprint.
B. Limited Access to Quality Data
AI systems rely on high-quality, real-time data for training and deployment. In many developing regions, data on climate patterns, energy use, or environmental changes may be incomplete, outdated, or unavailable.
- Impact: This limits the effectiveness of AI solutions in regions that are often the most vulnerable to climate change.
- Potential Solutions: Partnerships between governments, NGOs, and private companies can improve data collection and sharing, ensuring inclusivity in AI solutions.
C. High Initial Costs
Implementing AI-based climate solutions often requires significant upfront investment in technology, infrastructure, and skilled personnel. This can be a barrier for small organizations, startups, or developing nations.
- Impact: These financial barriers can delay the adoption of AI technologies in areas where they are most needed.
- Potential Solutions: Government subsidies, international funding, and public-private partnerships can make AI solutions more affordable.
D. Ethical and Privacy Concerns
The use of AI to monitor environmental and human activities can raise concerns about data privacy and surveillance. For instance, tracking deforestation using satellite data may inadvertently capture sensitive information about local communities.
- Potential Solutions: Transparent policies, ethical guidelines, and secure data-handling practices can address these concerns while maximizing AI’s benefits.
E. Risk of Overdependence on Technology
AI is a tool, not a replacement for systemic change. Over-reliance on AI solutions without addressing underlying issues like unsustainable consumption patterns or weak climate policies could undermine long-term progress.
- Potential Solutions: Combining AI with strong governance, community engagement, and education can ensure holistic climate action.
5. Future Prospects of AI in Climate Action (Enhanced)
The future of AI in climate change mitigation and adaptation holds immense potential. As technology advances, AI is expected to play a more significant role in creating innovative solutions.
A. Advancing Carbon Capture and Storage Technologies
AI can improve the efficiency and scalability of carbon capture and storage (CCS) systems by optimizing the capture process and identifying the best locations for storage.
- Example: Companies like Climeworks are already leveraging AI to enhance the performance of direct air capture systems.
- Future Outlook: AI could enable the widespread adoption of CCS, reducing emissions from industries that are difficult to decarbonize, such as cement and steel production.
B. Revolutionizing Smart Agriculture
AI-driven precision agriculture uses machine learning to analyze soil health, predict crop yields, and optimize water and fertilizer use.
- Example: John Deere’s AI-powered farming equipment helps farmers maximize yields while reducing resource waste.
- Future Outlook: By making farming more efficient and sustainable, AI can ensure food security while reducing agriculture’s carbon footprint.
C. Transforming Urban Planning
AI can support the design and management of smart cities by optimizing transportation, energy use, and waste management systems.
- Example: AI-powered traffic management systems, such as those used in Singapore, reduce congestion and emissions.
- Future Outlook: As urbanization increases, AI can help cities become more sustainable, reducing their environmental impact.
D. Predicting and Preventing Climate Disasters
AI models can analyze vast datasets to predict extreme weather events like hurricanes, floods, and droughts with greater accuracy. These predictions allow for better disaster preparedness and response.
- Example: IBM’s Watson AI is used to predict flooding patterns, enabling authorities to take preventive measures.
- Future Outlook: Integrating AI with IoT devices (e.g., smart sensors) will further enhance disaster forecasting and mitigation capabilities.
E. Renewable Energy Integration
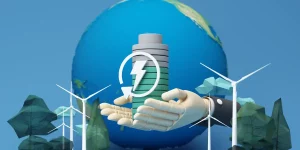
AI will play a central role in advancing renewable energy technologies. Machine learning models can predict energy production, balance supply and demand, and ensure the stability of energy grids.
- Example: Tesla’s AI-powered energy storage systems optimize the use of solar and wind power in real-time.
- Future Outlook: AI-driven energy solutions will make renewable energy more accessible, reliable, and cost-effective worldwide.
F. Enhancing Citizen Engagement
AI can help educate and engage citizens in climate action through interactive tools and platforms. For instance, AI-powered apps can provide individuals with actionable steps to reduce their carbon footprint.
- Example: Apps like Earth Hero use AI to suggest personalized eco-friendly actions.
- Future Outlook: Increased citizen engagement will amplify collective efforts to combat climate change.
Conclusion
The potential of AI to combat climate change is vast, with applications ranging from energy efficiency and disaster prediction to urban planning and agriculture. While challenges like energy consumption and data limitations persist, advancements in technology and collaborative efforts can address these hurdles. By integrating AI with robust policies and sustainable practices, we can harness its power to create a resilient and eco-friendly future. The time to act is now, and AI stands as a beacon of hope in the fight against climate change.
Artificial Intelligence is proving to be a game-changer in the fight against climate change. From improving energy efficiency to enhancing renewable energy systems and monitoring the environment, AI’s applications are vast and impactful. While challenges like energy consumption and data availability remain, the benefits far outweigh the drawbacks. By embracing AI, we can create a sustainable future and mitigate the impacts of climate change for generations to come.