Recent Breakthroughs in AI
The artificial intelligence industry has experienced significant advancements in the past few years, with ChatGPT occupying the spotlight. The most recent iteration, GPT-Four, possesses intelligence that is fifty times greater than earlier models. This can which creates high-quality text and proudly presents a user-friendly layout. Another pioneering progress is Reliable Spread. This allows converting text to images with the help of ML algorithms. These developments have brought about artificial intelligence more accessible to human existence. This raises inquiries into the potential and constraints. Nevertheless, even with their impressive abilities, ML models are still prone to shortcomings and partialities.
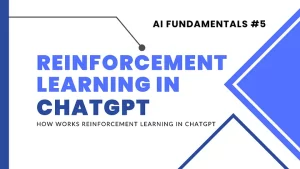
Reinforcement Learning in ChatGPT
Supervised learning becomes an important method to tackle the difficulties encountered by computational models. This is an algebraic system which enables analyzing various relationships and resolving everyday problems successfully. Within the framework of the ChatGPT system, RL has a vital importance in guaranteeing the well-being and consistency of the computational model.
Reinforcement Learning Introduction
Learning through reinforcement requires a robot interacting alongside a setting through action selection. The actions eventually cause the surroundings yielding a state together with a reward. The prize symbolizes the goal for maximizing, and the condition illustrates the present global arrangement. This individual uses a guideline to link circumstances to execute operations. The system learns to maximize reinforcement signals as time goes on disregarding where they come from. This limitless education empowers the individual to address intricate issues and adjust to different situations.
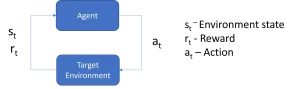
History of RL
The beginnings of learning through rewards are found in the act of deciding. Initial self-governing robots depended on non-artificial intelligence techniques to portray utility functions. An important achievement was achieved when AI systems employed a feedback model and a set of rules simultaneously. Society marked conduct as accurate or inaccurate. With reinforced learning through user input became popular, the mechanism of incentives and approach began to separate. This led to in the creation of higher-level technologies.
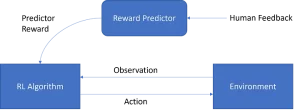
Reinforcement Learning with Human Feedback
The experiments conducted by OpenAI using Reinforcement Learning based on Human Feedback (RLHF) showed improvement when training models in the task of summarizing text. The findings indicated that RLHF has the ability to effectively improve the level of summarizing text. This included understanding written text, an assignment that individuals have executed in standardized evaluations for a considerable duration. Nonetheless, due to the progress of AI, machines are currently being created to execute equivalent tasks. The incorporation from user input in reinforcement learning with human feedback has brought about substantial breakthroughs in language understanding.
How to Train ChatGPT
Chatbot GPT, an influential RL application, altered the AI domain. This depends by utilizing RL algorithms to address user inquiries efficiently. Training procedures includes gathering immediate data sets and tagged outputs. This is followed by educating the incentive model and improving it using reinforcement learning. The incentive model ranks produced paragraphs, replicating human feedback, to boost the system’s performance.
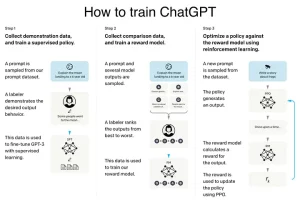
Internet Retail Platforms & Automated Fulfillment Robots by Amazon
Machine learning has usage within diverse areas, such as online marketplaces. It enables smooth communication amongst users and systems, boosting user experience and happiness. Moreover, Reinforcement Learning has played an important role in allowing Amazon’s automated warehouse robots to operate together with humans productively. This has assisted improve the efficiency of delivering packages and the management of storage operations, thus enhancing overall efficiency.
Model RL System
An all-inclusive RL system can be divided into three abstract parts. These parts are: Pretraining of Language Model, Reward Training, and Fine-tuning using Reinforcement Learning. Initial training entails constructing a language-based model that will be refined applying the reinforcement model. The compensation model outlines the bonus function for the reinforcement learning training. The procedure improves the language model’s efficiency, resulting in a more successful in actual use cases.
Clever Solutions inside the AI Sector
With the advancement of Reinforcement Learning keeps influencing the world of artificial intelligence, sophisticated solutions are coming up within multiple industries. Decision-making tools and prognostic models are becoming more trustworthy and exact. Due to The capability of RL to enhance choices based on observations.
Conclusion
RL has turned into a key aspect of AI. This guarantees the machine learning models such as ChatGPT work efficiently while ensuring safety and justice. Using RL’s flexible learning approach, Artificial Intelligence systems can tackle intricate problems and provide intelligent answers in diverse fields. These aid for producing people’s lives improved and improved productivity.