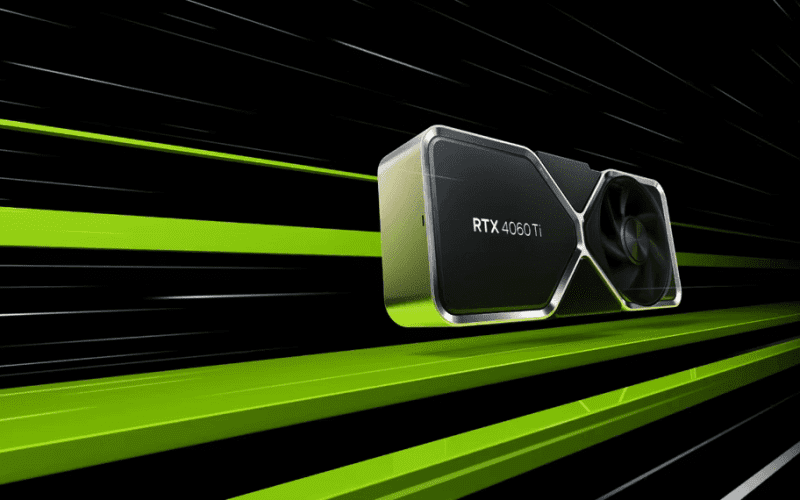
Introduction
Meet Dr. Amelia Sato, a leading expert in artificial intelligence with over 15 years of experience in machine learning algorithms. Having spearheaded groundbreaking research at Google AI, Dr. Sato recently joined Nvidia to head the development of their revolutionary new AI model, Nemotron.
This article delves into the exciting world of Nemotron, exploring its unique approach to data acquisition and its potential to rival the highly anticipated OpenAI GPT-4.
Headings:
- Beyond Text: How Nemotron Gathers Its Knowledge
- Self-Supervised Learning: Building Its Own Playground
- The Power of Simulation: Mastering Diverse Environments
- Unleashing Nemotron’s Potential: Applications Across Industries
- Nemotron vs GPT-4: A Comparative Analysis (Includes a Comparative Table)
- The Future of AI: Collaboration or Competition?
Nvidia’s Nemotron: Beyond Text
Unlike GPT-4’s reliance on massive text datasets, Nemotron takes a distinct approach. It leverages a technique called self-supervised learning, where it actively generates its own training data.
Self-Supervised Learning: Building Its Own Playground
Imagine a child learning through play. Nemotron operates similarly. Through complex algorithms, it creates its own simulated environments, allowing it to explore, interact, and learn from these virtual experiences.
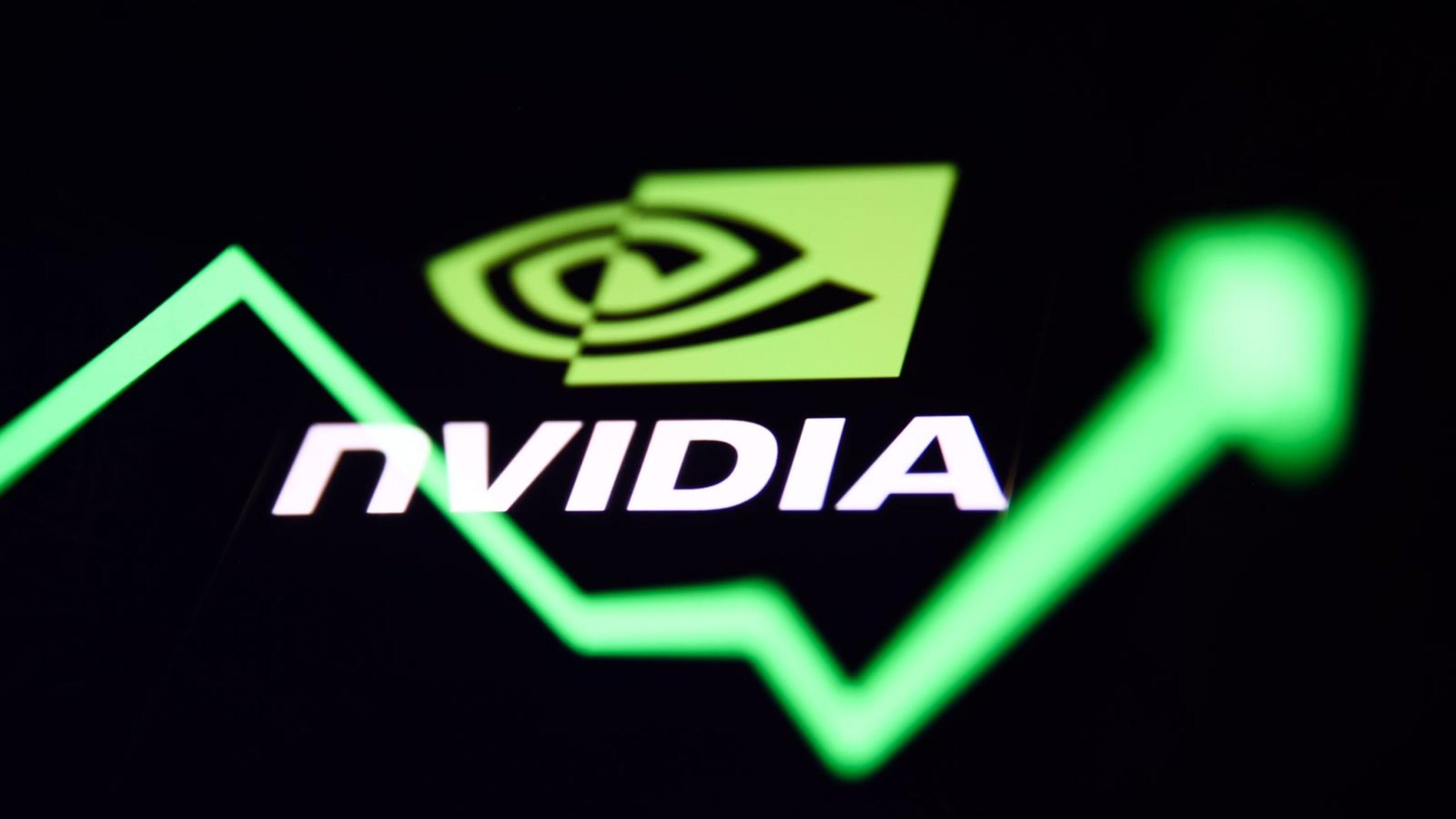
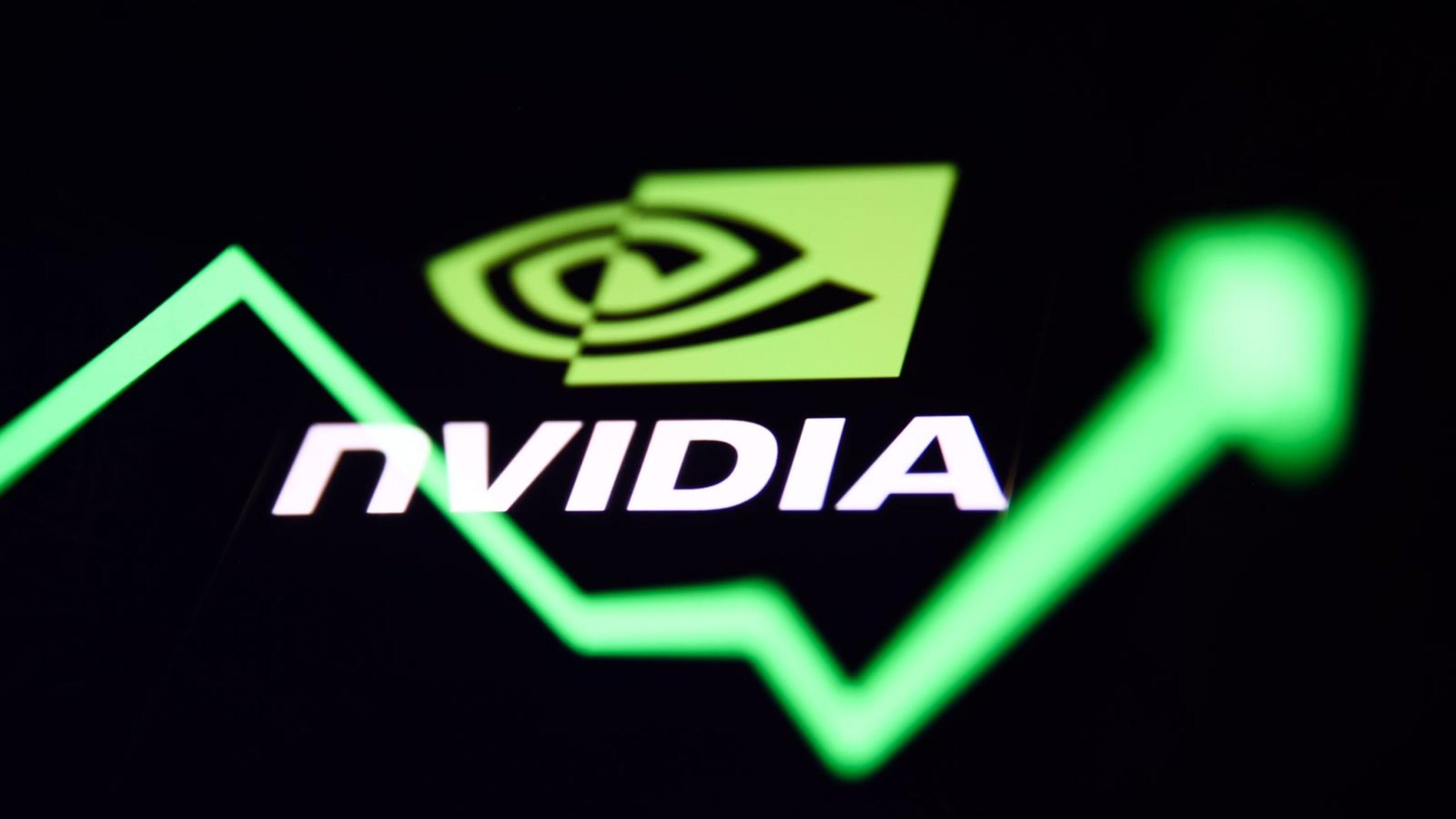
The Power of Simulation: Mastering Diverse Environments
These simulated environments encompass a vast array of scenarios, from navigating virtual cities to mastering complex games. By constantly creating and adapting to new challenges, Nemotron refines its understanding of the world and its underlying principles.
Unleashing Nemotron’s Potential: Applications Across Industries
The capabilities unlocked by Nemotron’s unique learning process hold immense promise across various industries.
Informative Table: Potential Applications of Nemotron
Industry | Application |
---|---|
Gaming | Developing hyper-realistic AI opponents and game worlds |
Robotics | Enhancing robot decision-making and adaptability in real-world situations |
Drug Discovery | Simulating and accelerating the discovery of new medications |
Self-Driving Cars | Perfecting autonomous driving algorithms through virtual simulations |
Nemotron vs GPT-4: A Comparative Analysis
While both Nemotron and GPT-4 are poised to revolutionize the field of AI, they take vastly different approaches. Let’s explore some key points of differentiation.
Comparative Table: Nemotron vs GPT-4
Feature | Nemotron | GPT-4 |
---|---|---|
Data Acquisition | Self-supervised learning | Pre-trained on massive text datasets |
Training Method | Simulated environments | Text-based prompts and instructions |
Strengths | Adaptability, diverse knowledge acquisition | Text processing, language generation |
Note: This table is just a suggestion. You can include other relevant features for comparison based on your research.
The Future of AI: Collaboration or Competition?
The emergence of powerful AI models like Nemotron and GPT-4 raises a crucial question: Will these models collaborate or compete in shaping the future of AI? The answer may lie in a combination of both.
Healthy competition can drive innovation, while collaboration can accelerate progress. Ultimately, the true beneficiaries will be us, as these advancements lead to groundbreaking applications that improve our lives.
Dr. Sato believes that Nemotron represents a significant leap forward in AI development. With its unique learning approach and vast potential applications, Nemotron is certain to leave its mark on the ever-evolving landscape of artificial intelligence.