Multi-Agent Reinforcement Learning: Methods, Applications, Prospects, and Challenges
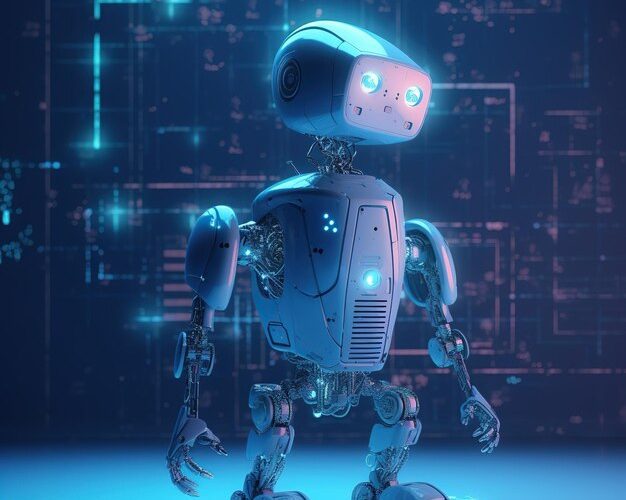
Introduction
Numerous applications of Reinforcement Learning have displayed notable potential in tackling sequence decision tasks in different fields. Various fields like game playing and robotics have thoroughly examined this area. Many agents are used in MARL to optimize their potential rewards. Though significant success has been attained, scaling, non-stationarity, and credibility remain significant issues. We offer a comprehensive review of MARL methods, application scenarios, and prospects in this paper. Emphasizes the necessity of reliable MARL and examines its utilization in human-machine interaction, discussing the difficulties related to it.
Methods
The article introduces SARL and MARL, which are both single and multi-agent reinforcement learning approaches. To maximize the potential total discounted reward, SARL focuses on learning an optimal policy. Describes the yearly rainfall levels of various cities and introduces Deep Q-Network and Policy Gradient as prominent methods in this work.
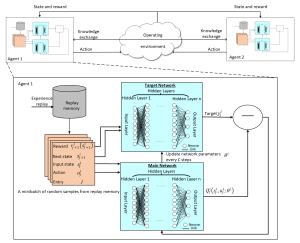
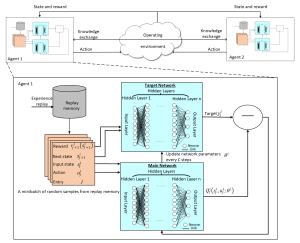
MARL Applications across multiple agents are presented in this table.
The publication showcases diverse scenarios where MARL has been effectively implemented. The applications across multiple domains including smart transportation, education, manufacturing, and security are showcased here.
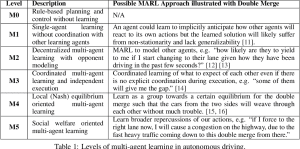
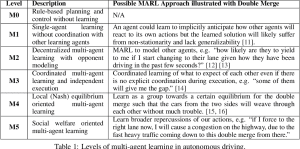
Prognostic prospects for multi-agent reinforcement learning.
The paper examines visionary prospects for MARL and emphasizes the importance of reliable MARL in practical applications. It explores safety, robustness, generalization, and learning with ethical constraints as core concepts of trustworthy MARL.
Examining the obstacles surrounding the enhancement of multi-agent reinforcement learning from human viewpoint.
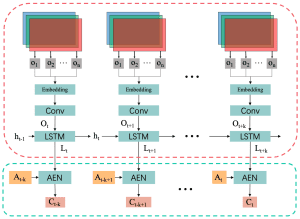
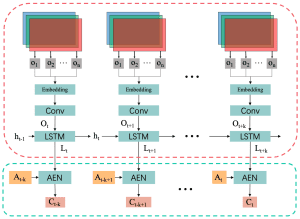
To understand human-MARL challenges in various interaction scenarios, the paper delves in. Analyzes non-Markovian human behavior due to external factors, diversity of human behavior, complexity of interactions, and scalability among multiple humans and machines.
Conclusion
The article summarizes the reviewed techniques, applications, and challenges in Multi-Agent Reinforcement Learning. Building trustable MARL frameworks is emphasized to ensure success in diverse applications. The significance of taking human interaction into account when developing MARL to enhance future interactions is emphasized.