Leveraging Transfer Learning to Enhance Data Quality: ChatGPT’s Approach
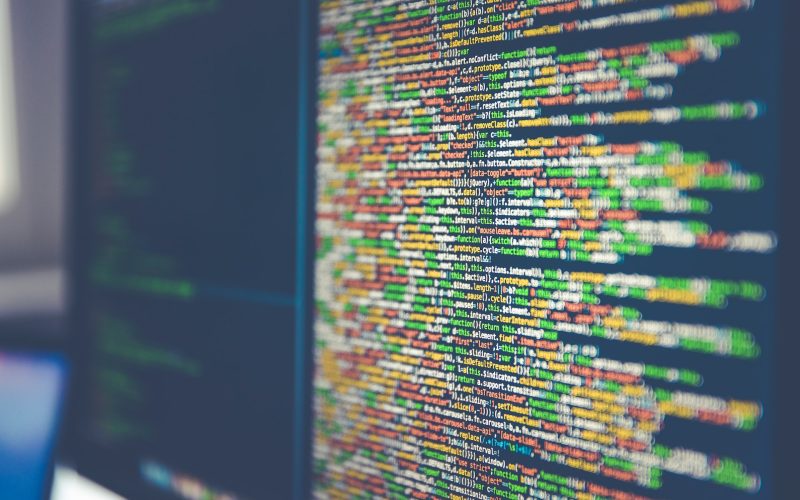
ChatGPT and the Use of Learning Transfer for Enhanced Data Accuracy
Machine learning (AI) platforms have advanced remarkable progress during recent times. A significant important developments fueling their achievements involves transfer learning. Knowledge transfer is a method that enables Artificial intelligence models to utilize knowledge attained from one task and utilize it to a different. These outcomes for quicker and optimized instructional techniques. Amidst the Artificial intelligence platforms utilizing this strategy is ChatGPT AI. It is a NLP (NLP) platform that aims to optimize data quality and optimize its artificial intelligence-powered chatbot through knowledge exchange.
Transforming Artificial Intelligence Training using Learning Transfer
Standard AI training requires training a model from the ground up. The procedure necessitates a substantial volume of information and computer resources. Transfer knowledge, however, enables developers to employ pre-existing models trained on massive datasets for diverse tasks. This serves as a groundbreaking within the field of AI training.
ChatGPT, designed by TS2 Space, takes advantage of transfer learning to optimize its chatbot’s performance and accuracy. Rather than initiating with arbitrary values and rigorous practice, ChatGPT can rely on understanding already obtained from a massive pool of discussions involving humans. This enables it to possess a deeper comprehension of language and situation, increasing its efficacy at generating reactions. This not just speeds up training and additionally boosts the chatbot’s knowledge of context. This results to increased exact and contextually fitting replies.
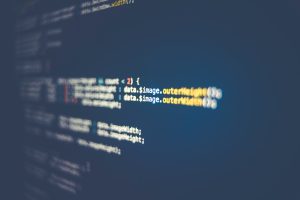
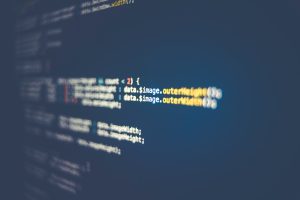
Increasing ChatGPT’s Precision Via Transfer Learning
One the main advantages through transfer learning within ChatGPT results in the enhancement in its precision. The system’s ability to a large collection of dialogues among human beings offers valuable information. These records can be employed via transfer learning. Through utilizing the knowledge already present, ChatGPT has the ability to more effectively understand the intricacies of language. This can further provide very specific replies to user questions.
Moreover, knowledge transfer facilitates ChatGPT to boost the precision of its data. Through identifying and removing mistakes in the current datasets, the application can verify that the dataset it utilizes for training is exact and consistent. This not just improves the efficiency of the AI bot but also guarantees an elevated degree of user contentment.
ChatGPT: A Robust Platform for Knowledge Transfer Scenarios
The architecture of ChatGPT, built on the GPT-3 model, offers a robust and versatile solution for multiple transfer learning applications. Nevertheless, it is worth mentioning that transfer learning is not universally applicable and might need adjustments for particular assignments. The capability to rapidly create top-notch models without needing extensive training data repositories it separates it from conventional methods. That is especially important during the time and materials are restricted.
In addition, ChatGPT’s ability to support multiple languages improves its capabilities to a greater extent. This model can produce content in different languages. This creates it valuable for software that demand language variety. The ease of inclusion in current applications makes the platform a compelling option for programmers looking to enhance their undertakings utilizing transfer learning abilities.
Investigating the Prospect of Learning Transfer employing ChatGPT
Transfer of learning has quickly gained popularity in the NLP industry. ChatGPT represents one of its own most encouraging utilizations. Through the reuse of pre-existing models, ChatGPT can speedily build interactive AI systems with little to no effort. The AI’s versatile capability empowers it to comprehend and react to various subjects. This creates it an adaptable device for different uses.
Furthermore, The capability of ChatGPT to gather knowledge from user engagements separates it from unchanging models. While the system progresses to engage with individuals, it grows increasingly knowledgeable during time period. This process learns through past events and enhances its replies. This flexible learning ability makes ConversationGPT a perfect instrument for developing AI systems that advance and get more advanced via each communication.
Utilizing knowledge transfer has played a key role in raising the data quality of ChatGPT. Through acquiring knowledge from a broader and varied dataset, the model develops a deeper comprehension of linguistic subtleties. It results to enhanced precision and contextually fitting replies. The capacity to utilize existing information from a massive data set enables ChatGPT to acquire at a faster pace and generate higher-quality outputs. This speeds up the formation of advanced chatbot systems.
Examining the Influence of Learning Transfer involving ChatGPT Efficiency
Current study regarding the influence associated with learning transfer concerning how well ChatGPT performs has delivered promising conclusions. A single study showed that knowledge transfer enhanced the performance of ChatGPT during a dialogue test resulting in a 25% improvement. This major improvement was credited to the model’s capacity to grasp more effectively the conversational context. That was able to also create more realistic responses.
The results of this study highlight the capability through knowledge transfer in relation to language models, notably in interactive platforms. Through utilizing information from different tasks, Artificial Intelligence developers can develop stronger and realistic conversational systems. It can result for better communication among humans and machines, enhancing user experience and empowering a larger scope of practical applications. This progress may lead a path to increasingly captivating and genuine dialogues between human beings and technology.
Conclusion
Knowledge transfer has demonstrated to be a revolutionary approach within the AI industry. In the case of platforms such as ChatGPT, it has created fresh opportunities for boosting data quality and strengthening AI-enhanced chatbots. Utilizing expertise from a single assignment to improve efficiency in a different one, knowledge transfer allows ChatGPT to have a better understanding of the context, generate more exact reactions, and acquire information from user exchanges. This creates it an effective tool for creating voice recognition systems. With the domain in Computational Linguistics is continuously improving. Transfer knowledge is expected to be instrumental in driving AI systems for increased efficiency and intelligence.