Introduction
Reinforcement learning (RL) Artificial Intelligence has witnessed the rise of RL as a potent paradigm.. By allowing agents to learn from their surroundings and optimize rewards through experimentation. Rather than relying solely on supervision, RL involves direct interaction with the environment which allows it to be applied in diverse areas such as robotics, natural language processing,and finance. The impact of RL is evident which include revolutionary breakthroughs such as AlphaGo, chatGPT, and optimized treatment plans for chronic disorders As RL undergoes constant development, keeping abreast of the latest advancements in this field is crucial for professionals and researchers. We will examine a few of the top (mainly complimentary) offerings accessible on the internet, encompassing tutorial videos, educational classes or programs,scholarly works along with others Supplemental materials can enhance your proficiency in reinforcement learning.
Online Courses
Reinforcement Learning Specialization – by Coursera
Offered by the University of Alberta and Alberta Machine Intelligence Institute incorporates RL fundamentals, value-focused methods, policy gradient methodologies, and deep reinforcement learning. Hands-on programming assignments and a final project enrich the way you learn.
Reinforcement Learning Lecture Series 2021 - by DeepMind x UCL
Offered by DeepMind and UCL, this series discusses core RL concepts, dynamic programming, learning without a model, deep reinforcement learning, and additional topics. Ideal for those interested in the latest advancements in RL, both researchers and practitioners.
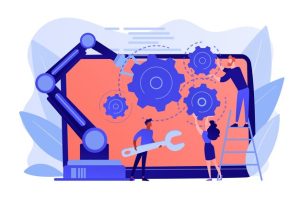
Stanford CS234: Reinforcement Learning – Winter 2019
Taught by Prof. Emma Brunskill, this course examines MDPs, MC methods, Temporal Difference-learning, and deep reinforcement learning. Ideal for individuals who have experience in machine learning and want to explore advanced techniques seeking advanced techniques.
Introduction to Reinforcement Learning with David Silver
An Overview of Reinforcement Learning presented by the distinguished speaker, David Silver with David Silver
With Prof. David Silver as their leader, one of the primary contributors to the advancement of AlphaGo. This course covers dynamic programming, Monte Carlo methods, exploration-exploitation trade-offs, and deep RL.
UC Berkeley CS 285: Deep Reinforcement Learning – Fall 2021
Centering around deep learning techniques in RL, offered to graduate students, taught by Prof. Sergey Levine covers Markov decision processes, deep Q-learning, policy gradient methods.
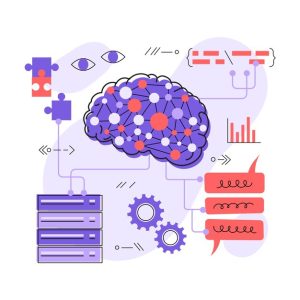
Deep RL BootCamp – UC Berkeley
Guided by esteemed scientists, this two-day course covers value-based and policy gradient methods, model-based RL, exploration and uncertainty. Moreover, it encompasses utilizing RL for real-world applications.
The Deep Reinforcement Learning Course provided brought to you by HuggingFace by HuggingFace
The content of this interactive course includes a comprehensive study of Q-learning methods, policy gradients algorithms,guidance on exploration strategies ,as well as applications of multi-agent RL and meta-learning. Accessible to all levels are practical projects and theoretical explanations Provide accessibility to people of all skill levels.
Lectures by Pieter Abbeel
Prof. Pieter Abbeel’s lectures on YouTube Prof. Pieter Abbeel presents valuable insights into RL ,apprenticeship leanring ,and open-source software develoment. for robotics and machine learning.
Spinning Up in Deep RL by OpenAI
OpenAI has developed this resource that offers a thorough introduction to deep RL, Included in this resource are algorithms, implementations, and papers The perfect opportunity to explore RL.
Phil Tabor’s RL Courses
Machine learning engineer Phil Tabor’s YouTube channel and Udemy courses present practical, interactive methods for RL, such as Q-learning algorithms, policy gradients techniques, and many others.
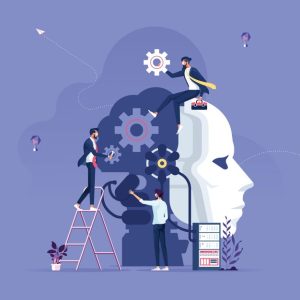
Books
Reinforcement Learning: An Introduction (Second Edition) – written by Richard Sutton and Andrew Barto
An indispensable resource for anyone enthusiastic about RL, this book covers foundational algorithms, deep RL, applications in robotics, game playing, healthcare, while considering the upcoming advancements and potential future developments in RL.
Decision Making Under Uncertainty: Theory and Application – by Mykel J. Kochenderfer
This book focuses on RL in decision-making under uncertainty, including topics such as planning, safe RL, and applying these techniques to fields like robotics and surveillance
Reinforcement Learning – by Phil Winder
A deep dive into RL fundamentals, Exploring Q-learning and policy gradient algorithms, and advanced topics like multi-agent RL and exploration.
The book titled, Deep Reinforcement Learning in Action, written by Alexander Zai and Brandon Brown
This hands-on guide explores DRL techniques, covering topics like DQN, actor-critic algorithms, and employing RL in practical scenarios.
Deep Reinforcement Learning Hands-On - by Maxim Lapan
Modified for DRL, The contents of this book encompass the fundamentals, DQN algorithm, policy gradient techniques, A3C implementation details, exploration methodologies as well as advanced subjects like AlphaGo Zero.
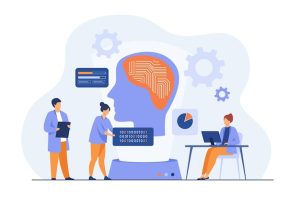
Bonus: Other Useful Resources
Article on on “The Best RL Tools in Python” Article by neptune.ai
GitHub repository “awesome-deep-rl” containing a curated list of deep RL materials.
A discussion on unleashing the power of Deep Reinforcement Learning for financial applications discussing RL’s applications in finance.
Conclusion
Artificial intelligence’s future is being influenced by reinforcement learning, with utilization in diverse sectors. With a plethora of online resources like courses, books, and additional materials, Accessing RL learning material has become incredibly convenient. If you’re just starting out or already have experience, You will gain the necessary knowledge to excel in the thrilling realm of reinforcement learning. Seize the chance to delve into RL and uncover its capacity to revolutionize AI applications.