Big Data has become a game-changer for industries worldwide, particularly in Data is Transforming the financial sector. It is reshaping the way financial institutions identify, assess, and manage risks. In this article, we’ll explore how Big Data is revolutionizing risk management in finance, providing clear examples, insights, and comparative analyses to showcase its transformative impact.
Understanding Big Data in Risk Management
What is Big Data?
Big Data refers to the massive volumes of structured and unstructured data generated daily. In the financial world, this data comes from various sources, including transaction records, market trends, social media analytics, and even customer behaviors.
Risk Management in Finance
Risk management involves identifying potential risks, measuring their impact, and developing strategies to mitigate them. Financial institutions face risks like credit risk, market risk, operational risk, and regulatory risk.
The Convergence of Big Data and Risk Management
By integrating Big Data technologies, financial institutions can now process and analyze vast amounts of data in real-time. This helps in better predicting and mitigating risks, offering a more proactive rather than reactive approach.
Key Applications of Big Data in Risk Management
1. Fraud Detection and Prevention
Big Data enables institutions to analyze transactional patterns and identify anomalies, which are often indicators of fraudulent activity. Machine learning algorithms enhance fraud detection by continuously improving their ability to recognize suspicious behavior.
- Example: Credit card companies use Big Data to flag transactions occurring in different locations within short intervals, which might indicate card theft.
2. Credit Risk Assessment
Traditional credit risk assessments relied heavily on historical data and credit scores. Big Data adds layers of granularity by analyzing alternative data sources such as social media activity, online behavior, and payment history.
- Example: Fintech firms use Big Data to provide loans to individuals with limited credit history by Data is Transforming assessing their financial habits.
3. Market Risk Analysis
Big Data analytics helps in tracking global market trends and predicting market volatility. This aids in informed decision-making and the development of risk-averse strategies.
- Example: Hedge funds utilize Big Data to analyze stock performance trends and mitigate potential losses during market downturns.
4. Regulatory Compliance
Regulatory requirements are becoming increasingly stringent. Big Data simplifies compliance by automating data collection, reporting, and analysis, reducing the likelihood of non-compliance penalties.
- Example: Automated systems powered by Big Data track regulatory changes and update risk protocols in real-time.
Benefits of Big Data in Risk Management
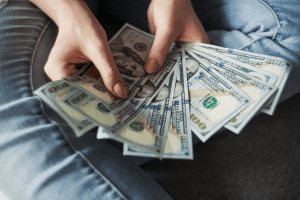
Enhanced Predictive Accuracy
Big Data improves the accuracy of risk predictions by analyzing diverse datasets and identifying patterns invisible to traditional methods.
Cost Efficiency
Automating risk management processes reduces manual labor and operational costs, making the financial system more efficient.
Real-Time Insights
Big Data systems provide real-time alerts and updates, enabling quick responses to potential risks.
Improved Customer Trust
By effectively managing risks and ensuring compliance, institutions build trust with their customers, enhancing brand reputation.
Challenges of Implementing Big Data in Finance
Data Privacy Concerns
Handling sensitive financial data raises concerns about privacy and cybersecurity. Institutions must adopt Data is Transforming robust security measures.
High Implementation Costs
Integrating Big Data technologies requires significant investment in infrastructure, software, and expertise.
Data Overload
Processing and analyzing massive datasets can overwhelm systems if not managed properly.
Talent Shortage
The demand for skilled data analysts and Big Data professionals often exceeds supply, creating a talent gap.
Comparative Analysis: Traditional vs. Big Data Risk Management
Aspect | Traditional Risk Management | Big Data Risk Management |
---|---|---|
Data Sources | Limited (historical financial data) | Diverse (social media, transactions, IoT data) |
Response Time | Reactive | Proactive and real-time |
Accuracy | Moderate | High (predictive analytics) |
Cost Efficiency | Labor-intensive, costly | Automated, cost-effective |
Scalability | Limited | Highly scalable with cloud-based solutions |
Analytical Insights Table
Key Area | Big Data Contribution | Impact on Risk Management |
---|---|---|
Fraud Detection | Anomaly detection through pattern recognition | Reduced fraudulent activities |
Credit Scoring | Alternative data analysis | Expanded access to credit |
Market Prediction | Predictive modeling based on trends | Improved investment decisions |
Compliance Monitoring | Automated tracking of regulations | Minimized regulatory penalties |
Future of Big Data in Financial Risk Management
The role of Big Data in risk management will continue to evolve with advancements in technologies like artificial intelligence, machine learning, and blockchain. As institutions adopt these innovations, risk management processes will become even more sophisticated, efficient, and secure.
Big Data’s Role in Proactive Risk Identification
Traditionally, financial institutions identified risks only after significant damage had occurred. Big Data transforms this approach by enabling real-time risk identification. With the ability to process vast amounts of structured and unstructured data, financial institutions can now identify subtle patterns that signal potential risks. For instance, analyzing a combination of market trends, geopolitical events, and customer transactions can provide early warnings about potential Data is Transforming market disruptions or economic downturns. This proactive approach allows companies to devise mitigation strategies in advance, ensuring a more robust financial framework that withstands shocks.
Integrating Machine Learning with Big Data in Finance
Machine learning is a critical enabler of Big Data analytics in finance. It helps institutions create predictive Data is Transforming models that evolve with time. Algorithms learn from historical and real-time data to detect emerging risks, such as sudden market volatility or an increase in fraudulent activities. For example, in the credit risk domain, machine learning models can evaluate an individual’s creditworthiness by considering non-traditional data points, like spending habits and social media activity. This integration reduces human bias, enhances accuracy, and opens up opportunities for inclusive financial services by catering to those without extensive credit histories.
Improving Stress Testing and Scenario Analysis
Stress testing and scenario analysis are vital components of risk management in finance, helping institutions prepare for worst-case scenarios. Big Data adds a layer of precision to these exercises by considering a wider range of variables and interdependencies. For example, banks can simulate the impact Data is Transforming of unexpected regulatory changes or global economic crises on their portfolios using historical and predictive data. With more accurate insights, financial institutions can allocate resources effectively and maintain stability during turbulent times. This enhances their ability to safeguard investor interests while complying with regulatory requirements.
Transforming Operational Risk Management
Operational risks, such as system failures, cyberattacks, and human errors, have become a significant concern for financial institutions. Big Data enhances operational risk management by providing a comprehensive view of potential vulnerabilities. For instance, by analyzing server logs, email patterns, and employee behavior, Big Data tools can flag unusual activities indicative of cyber threats or internal fraud. Similarly, predictive maintenance powered by Big Data ensures that critical systems remain functional, reducing downtime and avoiding costly disruptions. This capability not only saves costs but also ensures the continuity of essential financial services.
Revolutionizing Customer-Centric Risk Solutions
Big Data enables financial institutions to adopt a more customer-centric approach to risk management. By analyzing customer behavior and preferences, institutions can offer personalized risk solutions, such as tailored insurance products or customized investment portfolios. For instance, Data is Transforming by understanding a customer’s spending patterns, institutions can detect unusual activity and alert them promptly, thereby preventing fraud. Similarly, predictive analytics can help institutions design financial products that align with customers’ risk appetites, improving customer satisfaction and loyalty. This shift towards personalization highlights the role of Big Data in creating value beyond traditional risk management practices.
The Ethical and Regulatory Implications of Big Data Usage
As Big Data becomes central to risk management, ethical and regulatory concerns gain prominence. The use of Data is Transforming sensitive customer data raises questions about privacy and consent. Institutions must ensure that their data collection and usage practices comply with regulations like the General Data Protection Regulation (GDPR) or similar laws in other regions. Additionally, there is a need for transparency in how algorithms process data to make decisions, as opaque systems can lead to biases and unintended consequences. Addressing these challenges requires a balanced approach that prioritizes ethical considerations while leveraging Big Data for innovation in risk management.