Big data has become a game-changer in the retail industry, reshaping traditional business models and strategies through advanced analytics. This article explores the transformative impact of big data on retail analytics, enhancing customer insights, operational efficiencies, and competitive advantage.
1. Introduction to Retail Analytics
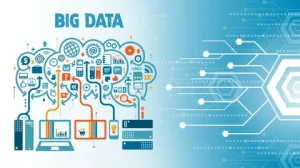
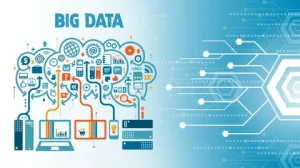
Retail analytics involves the use of data analysis tools and techniques to derive meaningful insights from customer behavior, sales trends, inventory management, and marketing effectiveness. Big data technologies have exponentially increased the volume, velocity, and variety of data available for analysis, driving deeper understanding and informed decision-making.
2. Customer Behavior Analysis
Big data enables retailers to analyze vast amounts of customer data collected from multiple touchpoints, including online platforms, mobile apps, and in-store interactions. By understanding purchasing patterns, preferences, and demographics, retailers can personalize marketing campaigns, optimize product offerings, and enhance customer experiences.
3. Predictive Analytics for Demand Forecasting
Predictive analytics leverages historical sales data, external factors like weather and economic trends, and real-time data streams to forecast future demand accurately. Retailers can optimize inventory levels, reduce stockouts, and improve supply chain efficiency by aligning production and distribution with anticipated demand fluctuations.
4. Enhancing Operational Efficiency
Big data analytics streamlines operational processes across retail operations, from inventory management to logistics and workforce optimization. Real-time analytics enable agile decision-making, improving resource allocation and reducing operational costs while maintaining service quality and customer satisfaction.
5. Personalized Marketing Strategies
Retailers use big data to create personalized marketing strategies based on individual customer preferences and behaviors. Targeted advertising, product recommendations, and tailored promotions enhance customer engagement, loyalty, and ultimately, sales conversion rates.
6. Omnichannel Integration
Omnichannel retailing integrates online and offline customer interactions seamlessly. Big data analytics unify data from various channels, providing a holistic view of customer journeys and enabling retailers to deliver consistent experiences across touchpoints, whether in-store, online, or via mobile devices.
7. Fraud Detection and Security
Big data analytics tools monitor transactions and customer behavior patterns to detect anomalies indicative of fraudulent activities. Advanced algorithms analyze real-time data streams to mitigate risks, safeguard customer data, and protect retailers from financial losses and reputational damage.
8. Competitive Intelligence
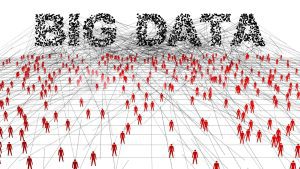
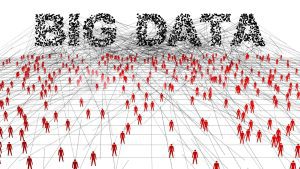
Big data enables retailers to gain insights into competitor strategies, pricing trends, and market dynamics. Analyzing external data sources such as social media trends and industry reports empowers retailers to identify opportunities, anticipate market shifts, and stay ahead of competitors in a rapidly evolving landscape.
9. Challenges in Implementing Retail Analytics
Despite its benefits, implementing effective retail analytics poses challenges such as data integration complexities, data privacy concerns, and the need for skilled data analysts. Overcoming these hurdles requires robust data governance frameworks and investment in talent and technology infrastructure.
10. Future Trends in Retail Analytics
The future of retail analytics lies in leveraging emerging technologies like artificial intelligence and machine learning to enhance predictive capabilities and real-time decision-making. Anticipated developments include AI-driven customer service bots, hyper-personalized shopping experiences, and enhanced data-driven supply chain management.