In today’s increasingly digital world, cybersecurity has become one of the most critical concerns for organizations across all industries. With the rise of cyber threats that grow more sophisticated every year, traditional cybersecurity methods, which often rely on manual processes and signature-based detection systems, are no longer enough to protect sensitive data and systems. Enter Artificial Intelligence (AI)—a transformative technology that is revolutionizing the way threats are detected and mitigated.
AI’s ability to process large volumes of data, recognize patterns, and make decisions in real-time has proven invaluable in the cybersecurity space. It empowers organizations to not only detect threats more efficiently but also predict and prevent potential attacks before they happen. This article explores how AI is transforming threat detection in cybersecurity, its benefits, real-world examples, and the future of AI-powered security systems.
The Need for AI in Threat Detection
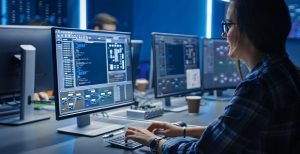
Before diving into how AI is transforming cybersecurity, it’s important to understand the growing complexity of cyber threats. Cyberattacks today are more advanced, targeted, and persistent than ever. Traditional methods of detecting malware and intrusions often fail to identify new, evolving, or polymorphic threats. Attackers are leveraging sophisticated tactics such as zero-day vulnerabilities, advanced persistent threats (APTs), and social engineering attacks to bypass conventional security defenses.
AI’s primary advantage lies in its ability to automate threat detection while continuously learning and adapting to new threats. Machine learning (ML) algorithms enable AI systems to analyze enormous datasets, identify patterns, and recognize anomalies in real time. This capability significantly enhances the accuracy and speed of threat detection, enabling organizations to respond quickly and effectively to potential risks.
AI-Driven Threat Detection Mechanisms
1. Anomaly Detection
One of the primary uses of AI in cybersecurity is anomaly detection. AI systems can establish a baseline of normal activity within a network or system, then continuously monitor for any deviations from this baseline. Anomalies can indicate malicious activities such as unauthorized access, data exfiltration, or the presence of malware. The key advantage of AI-powered anomaly detection is its ability to identify previously unknown threats that do not have known signatures.
For example, if a user typically accesses their company’s database during working hours, but an AI system detects an unusual login at 3 AM from an unfamiliar location, it can flag this as suspicious. This proactive approach helps detect threats that might otherwise go unnoticed by traditional security systems.
2. Behavioral Analytics
AI also leverages behavioral analytics to identify threats based on user and entity behavior. By tracking how individuals interact with systems, AI can detect irregularities that may suggest malicious intent. For instance, if an employee suddenly starts downloading large volumes of sensitive data or accessing systems they don’t usually interact with, AI can flag these actions as potentially harmful.
Behavioral analytics can also be used to detect insider threats, which are notoriously difficult to identify. These threats may involve employees or contractors exploiting their access privileges for malicious purposes. AI can continuously monitor user behavior to detect signs of insider activities, even if the user is authorized to access certain systems.
3. Machine Learning for Threat Classification
AI-powered machine learning models are capable of classifying threats based on their characteristics and behavior. These models are trained on vast datasets of known attacks and can recognize patterns that might indicate a new, previously unseen type of threat. By continuously learning from new data, these models become more accurate over time and are able to classify new attacks more effectively.
For example, AI can identify the difference between benign system activity and a potentially malicious file that behaves like a known piece of malware. This ability to classify threats accurately helps reduce false positives, which are a common issue with traditional signature-based detection systems.
4. Real-Time Threat Detection
Traditional cybersecurity systems often have a lag in detecting and responding to threats, sometimes allowing attacks to propagate before being identified. AI enables real-time detection by processing and analyzing data as it is generated. This capability is particularly critical for detecting time-sensitive threats like ransomware attacks, where swift action is needed to contain the damage before it spreads.
In addition to real-time detection, AI systems can prioritize threats based on severity, enabling security teams to focus their resources on the most urgent risks. For example, if an AI system detects a ransomware attack in progress, it can immediately alert security teams, isolate the affected system, and block any further malicious activity, all in real time.
Benefits of AI in Threat Detection
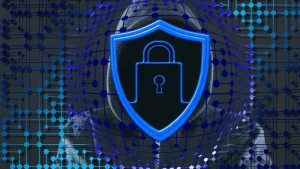
The integration of AI into threat detection systems offers several key benefits for organizations:
1. Enhanced Accuracy
AI-powered threat detection systems are more accurate than traditional methods because they rely on data-driven models and continuous learning. By analyzing large volumes of data, AI can identify subtle patterns that may be overlooked by human analysts or conventional tools. This leads to fewer false positives and more reliable threat detection.
2. Faster Response Time
The speed of AI-driven systems allows for faster detection and response to security incidents. AI can automatically flag suspicious activities, deploy countermeasures, and alert security teams within seconds, significantly reducing the time it takes to contain and mitigate threats. This quick response is essential in preventing data breaches, financial loss, or system damage.
3. Proactive Threat Detection
AI not only detects known threats but also anticipates potential risks by recognizing patterns that could indicate future attacks. This predictive capability allows organizations to implement preventive measures before an attack occurs, rather than waiting for a breach to happen.
4. Reduced Human Error
Cybersecurity professionals are often overwhelmed by the volume of alerts and incidents they must respond to. AI can handle much of the repetitive work, reducing the burden on human analysts and minimizing the risk of human error. By automating routine tasks such as log analysis and incident triage, AI frees up security teams to focus on more complex issues.
5. Scalability
AI-based systems can scale more easily to handle growing volumes of data, making them ideal for large enterprises or organizations with complex IT infrastructures. As cyber threats evolve, AI systems can continuously adapt to new challenges without requiring significant reprogramming or manual updates.
Examples of AI in Action: Case Studies
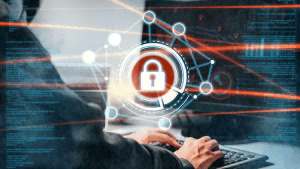
1. Darktrace and AI-Powered Cyber Defense
Darktrace, a leading provider of AI-driven cybersecurity solutions, uses machine learning to detect and respond to cyber threats autonomously. Darktrace’s Enterprise Immune System is an AI-powered security platform that continuously learns from the behavior of users and devices within an organization. It can detect even the most subtle signs of malicious activity and respond in real time.
For example, Darktrace successfully detected and contained a ransomware attack within minutes at a large healthcare organization. The AI system identified unusual file encryption patterns and immediately isolated the affected device, preventing the spread of the ransomware and saving the organization from potentially catastrophic data loss.
2. CrowdStrike and Predictive AI in Threat Hunting
CrowdStrike, a cybersecurity firm known for its advanced endpoint protection, uses AI and machine learning to enhance its threat-hunting capabilities. Their platform, Falcon, leverages AI to predict and detect cyber threats in real-time. By analyzing millions of endpoints and continuously learning from new data, Falcon can spot patterns that indicate potential attacks before they manifest.
In one case, CrowdStrike’s AI system detected a sophisticated APT (Advanced Persistent Threat) that had evaded other security measures for months. By analyzing behavioral patterns, Falcon was able to identify the threat and alert the security team, leading to a swift mitigation and preventing further damage.
3. IBM QRadar and AI-Enhanced SIEM
IBM’s QRadar is a Security Information and Event Management (SIEM) solution that integrates AI to enhance threat detection. By leveraging machine learning, QRadar can analyze vast amounts of security event data to identify unusual patterns and behaviors, allowing security teams to focus on the most critical threats.
QRadar’s AI-powered anomaly detection system was credited with uncovering a data breach in a financial institution’s network that had gone unnoticed by traditional security tools. The AI system flagged unusual access patterns in real-time, allowing the organization to respond and prevent further data loss.
Challenges and Considerations
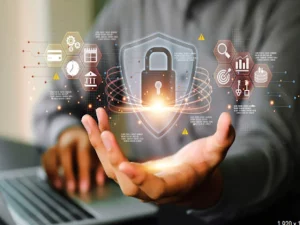
While AI offers tremendous benefits, there are also some challenges associated with its implementation in cybersecurity:
- Data Privacy and Security: AI systems require access to vast amounts of data to learn and make accurate predictions. Ensuring that this data is securely handled and does not introduce new vulnerabilities is crucial.
- Adversarial AI: Hackers may also use AI to launch more sophisticated attacks, such as adversarial machine learning, where they manipulate AI models to bypass security systems.
- Skill Gaps: Implementing and managing AI-powered cybersecurity systems requires a skilled workforce. Organizations may face challenges in recruiting and retaining cybersecurity experts with AI expertise.
Conclusion
AI is revolutionizing the way threats are detected and mitigated in cybersecurity. Its ability to process vast amounts of data, identify patterns, and make real-time decisions enables organizations to detect and respond to cyber threats with unprecedented speed and accuracy. As cyber threats continue to evolve, AI will remain an essential tool in the fight against cybercrime, helping businesses protect their data, systems, and reputations.
With its ability to detect unknown threats, predict potential risks, and automate incident response, AI is not just a reactive tool—it’s a proactive force in safeguarding the digital world. As AI technologies continue to improve, we can expect even more advanced and effective security systems to emerge, further enhancing the overall cybersecurity landscape.