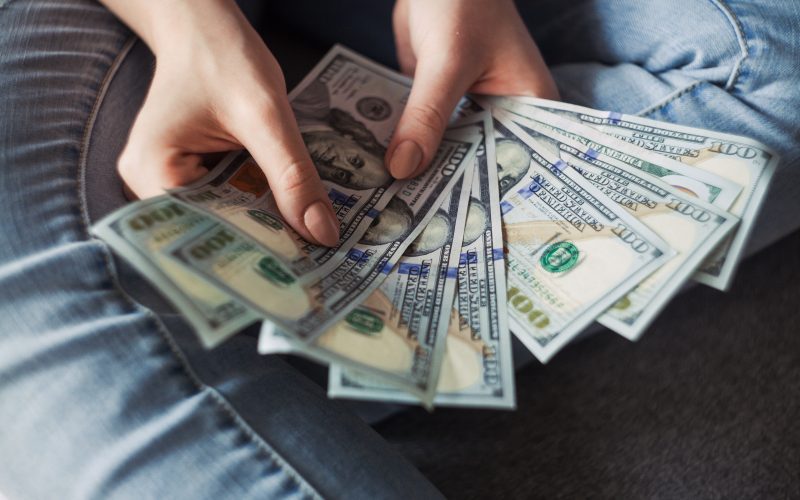
Fraud in financial services has become increasingly sophisticated, posing significant challenges for institutions and their customers. Traditional methods of fraud detection often fall short in identifying complex fraudulent activities. However, the advent of Artificial Intelligence (AI) has transformed the landscape of fraud detection, making it more effective and efficient. This article explores how AI is enhancing fraud detection in financial services, highlighting its benefits, technologies, and real-world applications.
Understanding Fraud in Financial Services
Fraud in financial services can take many forms, including credit card fraud, identity theft, money laundering, and insider trading. According to recent studies, financial fraud costs institutions billions of dollars annually, not to mention the reputational damage and loss of customer trust. As fraudsters develop new tactics, it is crucial for financial institutions to adopt advanced technologies to stay ahead.
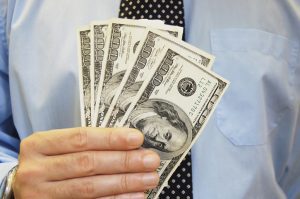
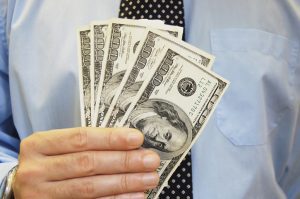
The Limitations of Traditional Fraud Detection Methods
Traditional fraud detection methods primarily rely on rule-based systems and manual reviews. While these systems can be effective for straightforward fraud cases, they often struggle with more complex schemes. Common limitations include:
- High False Positives: Rule-based systems frequently flag legitimate transactions as fraudulent, leading to customer dissatisfaction.
- Slow Response Times: Manual reviews can delay responses to suspicious activities, allowing fraud to occur.
- Inability to Adapt: Traditional systems may not learn from new fraud patterns, making them less effective over time.
The Role of AI in Fraud Detection
Artificial Intelligence, particularly machine learning (ML) and deep learning, has emerged as a game-changer in fraud detection. AI systems can analyze vast amounts of data in real-time, identifying patterns and anomalies that might indicate fraudulent activity. Here are some key ways AI enhances fraud detection:
1. Real-Time Data Analysis
AI algorithms can process and analyze transactions as they occur. By leveraging historical data and behavioral patterns, AI systems can identify potentially fraudulent transactions within seconds, significantly reducing the risk of losses.
2. Enhanced Accuracy
Machine learning models can learn from previous fraud cases and improve their predictive capabilities over time. This ability to adapt and evolve makes AI systems more accurate than traditional methods, reducing the incidence of false positives and ensuring legitimate transactions are not hindered.
3. Behavioral Analytics
AI can analyze customer behavior to establish a baseline of normal activity. Any deviation from this norm can trigger alerts for further investigation. For instance, if a customer suddenly makes a large purchase in a different geographic location, the AI can flag this transaction for review.
4. Network Analysis
AI can also identify fraud rings and networks by analyzing relationships between various accounts and transactions. This holistic view enables institutions to detect organized fraud schemes that may be missed by traditional methods.
Technologies Behind AI Fraud Detection
Several AI technologies are crucial for enhancing fraud detection in financial services. Here are the primary technologies involved:
Technology | Description | Applications |
---|---|---|
Machine Learning | Algorithms that improve over time by learning from data | Transaction monitoring |
Deep Learning | Advanced neural networks that can analyze unstructured data | Image and document verification |
Natural Language Processing (NLP) | Technology for understanding human language | Customer service chatbots for fraud inquiries |
Predictive Analytics | Techniques that forecast future outcomes based on historical data | Risk assessment and scoring |
Comparative Analysis of Traditional vs. AI Fraud Detection
The following table compares traditional fraud detection methods with AI-driven approaches, highlighting their differences and advantages.
Feature | Traditional Fraud Detection | AI Fraud Detection |
---|---|---|
Detection Speed | Slower due to manual processes | Real-time detection |
False Positive Rate | High, often leading to customer complaints | Lower, more accurate predictions |
Adaptability | Rigid, struggles with evolving fraud tactics | Learns and adapts to new patterns |
Data Processing | Limited to predefined rules | Analyzes vast datasets in real-time |
Resource Requirement | Labor-intensive and time-consuming | Automated, reducing the need for manual review |
Real-World Applications of AI in Fraud Detection
Many financial institutions are successfully implementing AI for fraud detection. Here are a few examples:
Case Study 1: American Express
American Express utilizes machine learning algorithms to analyze customer transactions in real-time. By continuously refining their models, they have significantly reduced fraud while enhancing the customer experience.
Case Study 2: PayPal
PayPal employs AI to monitor transactions across its platform. By leveraging neural networks, PayPal can identify unusual patterns indicative of fraud, protecting both buyers and sellers.
Case Study 3: HSBC
HSBC uses AI to analyze customer data and detect potential money laundering activities. Their systems can process vast amounts of information quickly, allowing them to comply with regulatory requirements more efficiently.
The Impact of AI on Customer Trust
One of the most significant benefits of AI in fraud detection is its positive impact on customer trust. When financial institutions use advanced technologies to safeguard transactions, customers feel more secure knowing that their assets are protected. By minimizing false positives and streamlining the approval process for legitimate transactions, AI enhances the overall customer experience. This improved trust can lead to increased customer loyalty and a stronger reputation for the institution, which is invaluable in a competitive market.
Collaborating with Regulators
As AI technologies evolve, financial institutions must work closely with regulators to ensure compliance and ethical use. Regulatory bodies are beginning to recognize the potential of AI in enhancing fraud detection but also emphasize the importance of transparency and accountability. By collaborating with regulators, financial institutions can create frameworks that not only protect consumers but also foster innovation. This partnership will help set industry standards for the responsible use of AI in fraud detection.
Continuous Improvement through Feedback Loops
Another advantage of AI in fraud detection is its ability to create feedback loops for continuous improvement. As new fraud patterns emerge, AI systems can adapt by analyzing the results of their predictions. By incorporating feedback from both successful detections and missed fraud cases, these systems become increasingly sophisticated. This ongoing learning process ensures that AI tools remain effective over time, allowing financial institutions to stay one step ahead of fraudsters. In this dynamic environment, the agility provided by AI is essential for maintaining robust fraud defenses.
Challenges in Implementing AI for Fraud Detection
While AI presents numerous benefits, implementing these technologies also poses challenges:
1. Data Privacy Concerns
Handling sensitive customer data raises privacy issues. Financial institutions must ensure compliance with regulations such as GDPR and CCPA.
2. Initial Costs
Implementing AI systems can require significant upfront investment in technology and talent. However, the long-term savings often outweigh these initial costs.
3. Integration with Existing Systems
Integrating AI solutions with legacy systems can be complex. Financial institutions need to ensure seamless interoperability to maximize effectiveness.
The Future of AI in Fraud Detection
As technology continues to evolve, the future of AI in fraud detection looks promising. Innovations such as quantum computing and advanced neural networks may further enhance detection capabilities. Additionally, as AI systems become more sophisticated, they will likely play an even greater role in ensuring the security of financial transactions.
Conclusion
AI is revolutionizing fraud detection in financial services by offering real-time analysis, improved accuracy, and the ability to learn from new data. While challenges exist, the advantages far outweigh the drawbacks. As financial institutions increasingly adopt AI technologies, they can better protect their customers and reduce the financial impact of fraud.