Algorithmic Bias and the Quest for Fairness in Machine Learning
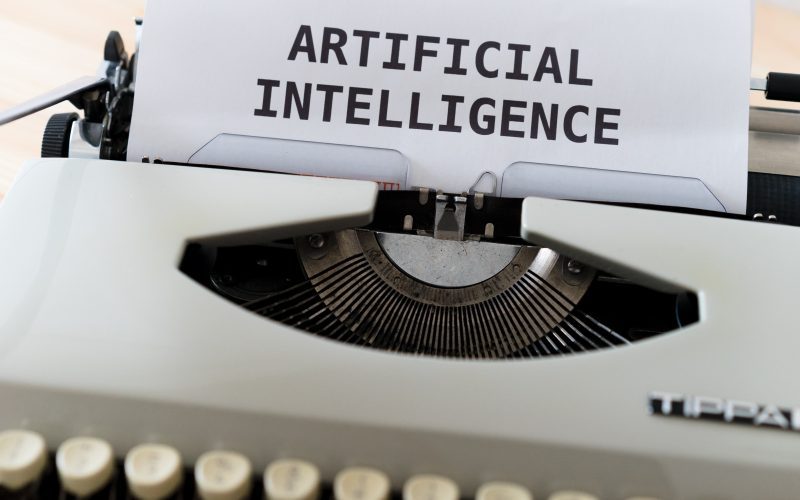
Machine learning algorithms have become an integral part of our lives, from personalized recommendations on social media to self-driving cars. However, these algorithms are not always fair, and can perpetuate biases that exist in society. This is known as algorithmic bias, and it is a growing concern in the field of machine learning.
What is Algorithmic Bias?
Algorithmic bias occurs when machine learning algorithms discriminate against certain groups of people based on their race, gender, age, or other characteristics. This can happen when the data used to train the algorithm is biased, or when the algorithm itself is designed in a way that perpetuates existing biases.
For example, a facial recognition algorithm may be trained on a dataset that is predominantly white, leading to inaccurate results for people with darker skin tones. Similarly, a hiring algorithm may be designed to favor candidates who attended prestigious universities, which can perpetuate socioeconomic biases.
Why is Algorithmic Bias a Problem?
Algorithmic bias can have serious consequences, particularly for marginalized communities. Biased algorithms can perpetuate discrimination and reinforce existing power imbalances. For example, a biased hiring algorithm could prevent qualified candidates from getting jobs, while a biased criminal justice algorithm could lead to unfair sentencing.
In addition to the ethical concerns, algorithmic bias can also have financial implications. Biased algorithms can lead to lost revenue and damage to a company’s reputation. For example, Amazon’s hiring algorithm was found to be biased against women, leading to a loss of trust among customers and potential legal action.
What is being done to address Algorithmic Bias?
There is growing recognition of the need to address algorithmic bias in machine learning. Researchers and practitioners are working to develop methods for detecting and mitigating bias in algorithms.
One approach is to ensure that the data used to train algorithms is diverse and representative of the population. This can involve collecting data from a variety of sources and ensuring that it is balanced in terms of race, gender, and other characteristics.
Another approach is to develop algorithms that are designed to be fair. This can involve using techniques such as counterfactual fairness, which involves adjusting the algorithm to ensure that it would have made the same decision regardless of the individual’s race, gender, or other characteristics.
There are also efforts to increase transparency and accountability in machine learning algorithms. This can involve making the algorithms and their decision-making processes more transparent, as well as providing avenues for recourse for individuals who have been harmed by biased algorithms.
Conclusion
Algorithmic bias is a complex and multifaceted problem that requires a concerted effort from researchers, practitioners, and policymakers to address. While there is no one-size-fits-all solution, there are a variety of approaches that can be taken to mitigate bias in machine learning algorithms. By working together, we can ensure that these algorithms are fair and equitable for all.