Facing Changes: Continual Entity Alignment for Growing Knowledge Graphs
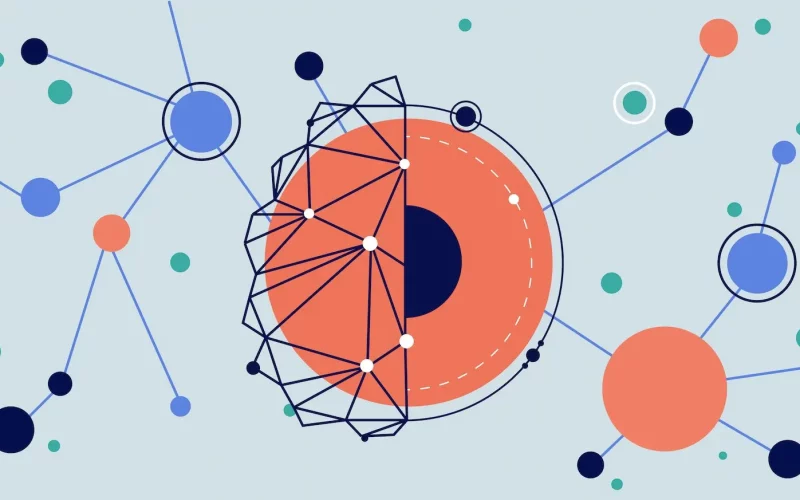
Introduction:
Entity alignment, a fundamental task in the Semantic Web and Database communities, involves matching identical entities with different URIs in various knowledge graphs (KGs). Traditional methods mainly relied on literal similarities, but recent embedding-based approaches introduced entity embeddings to achieve alignment. However, these methods often assumed static KGs, neglecting real-world challenges such as alignment incompleteness and KG growth. In this article, we present “continual entity alignment,” a novel approach that addresses these issues and enables alignment in evolving and incomplete KGs.
The Challenge of Entity Alignment in Growing KGs:
Knowledge graphs are dynamic entities that continuously expand and evolve through the addition and updating of data. This constant growth poses significant challenges for embedding-based entity alignment methods, as they must adapt to handle new entities efficiently and effectively. Additionally, capturing potential alignments between both old and new entities becomes crucial, and integrating old and new predictions necessitates careful consideration.
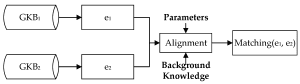
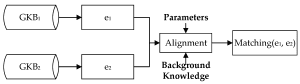
Introducing Continual Entity Alignment (ContEA):
Our proposed approach, ContEA, tackles continual entity alignment by fine-tuning pre-trained models to incorporate new entities and triples, capturing potential entity alignments, and integrating predictions from both old and new data.
The Key Components of ContEA:
ContEA leverages the Dual-AMN model as the basal encoder and introduces an entity reconstruction objective to handle new entities effectively. To retrieve alignments, we introduce a bidirectional nearest neighbor search strategy, ensuring more reliable alignment retrieval.
Datasets for Research on Continual Entity Alignment:
To facilitate research in this evolving setting, we construct three new datasets based on the widely-used benchmark DBP15K. Each dataset contains six snapshots, simulating KG growth by incorporating new entities and triples.
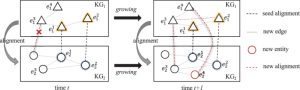
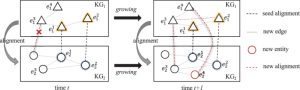
Experimental Results:
We conduct extensive experiments on our datasets, comparing ContEA with strong baselines that employ retraining or inductive embedding techniques. ContEA exhibits superior performance compared to the baselines while requiring less processing time.
Future Research Opportunities:
Continual entity alignment opens exciting avenues for enhancing alignment processes in dynamic KGs. Future research can explore various strategies for updating pre-trained models and improving alignment retrieval for new entities.
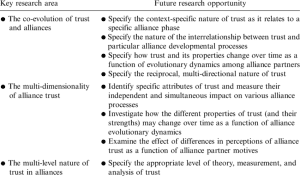
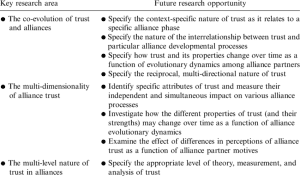
Conclusion:
Continual entity alignment emerges as a promising approach for matching entities in growing knowledge graphs. ContEA demonstrates its effectiveness in handling new entities, capturing potential alignments, and integrating predictions from both old and new data. As knowledge graphs continue to evolve, continual entity alignment will play a vital role in maintaining accurate and up-to-date entity alignments for diverse applications in the Semantic Web and beyond.