Environmental challenges, ranging from climate change to resource depletion, demand innovative solutions. Artificial intelligence (AI) and machine learning (ML) are revolutionizing environmental monitoring, offering new tools to address these pressing concerns. By enhancing accuracy, efficiency, and scalability, AI and ML are driving significant advancements across various domains.
Introduction to AI and ML in Environmental Monitoring
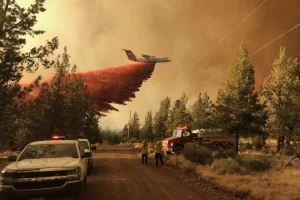
AI and ML technologies analyze vast datasets, identify patterns, and generate predictions with unmatched precision. Their application in environmental monitoring encompasses areas such as air and water quality, weather forecasting, and disaster management. The integration of these technologies is not just improving data processing but also transforming decision-making processes in environmental science.
Advancements in Wildfire Surveillance
Wildfires are a growing global threat, exacerbated by climate change. AI and ML have introduced innovative methods for wildfire detection and monitoring.
- Role of UAV Networks: Unmanned Aerial Vehicles (UAVs) equipped with federated learning (FL) and deep learning (DL) algorithms offer real-time surveillance. These systems outperform traditional methods, providing early detection and rapid response capabilities.
- Case Study: In California, AI-driven UAV networks were deployed to monitor wildfire-prone areas. These systems detected small fires before they spread, saving thousands of acres of forest and reducing response time significantly.
Water Quality Assessment Through AI
Monitoring water quality is critical for public health and environmental conservation. AI and ML algorithms have enhanced the ability to detect contaminants and predict water quality trends.
- Satellite-Based Monitoring: Sentinel-2 satellite data, combined with ML algorithms, monitors parameters like turbidity and chlorophyll levels in coastal waters. This scalable approach provides precise, timely assessments.
- Example: Researchers in Europe utilized AI to track harmful algal blooms, predicting their occurrence weeks in advance. This enabled proactive measures to mitigate their impact on marine ecosystems and fisheries.
AI-Driven Air Quality Prediction Models
Air quality monitoring has been revolutionized by AI, particularly in regions with limited infrastructure.
- Integration of Data Sources: AI models combine ground-based measurements with satellite data to predict pollutant distribution. These predictions help policymakers design effective interventions.
- Case Study: In India, AI-powered air quality models identified high-risk areas, allowing for targeted actions like traffic management and industrial emission controls. This led to a measurable reduction in pollution levels.
Revolutionizing Weather Forecasting with AI
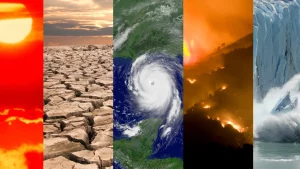
Accurate weather forecasting is crucial for agriculture, disaster management, and daily life. AI-driven models have transformed traditional forecasting methods.
- Introduction to GenCast: Google’s GenCast AI model leverages historical weather data to predict future patterns with high accuracy. It provides faster and more reliable forecasts, including early warnings for extreme events.
- Example: During Hurricane Milton, GenCast predicted the storm’s trajectory days ahead of conventional models, enabling better preparation and reducing potential damage.
AI in Natural Disaster Response and Management
Natural disasters pose significant risks to human lives and infrastructure. AI is enhancing detection, preparedness, and response strategies.
- Urban Disaster Detection Systems: AI systems analyze seismic data, flood patterns, and other indicators to provide early warnings. These systems are particularly effective in densely populated urban areas.
- Global Initiatives: Programs like the Global Initiative on Resilience to Natural Hazards through AI Solutions aim to standardize AI applications for disaster management.
- Case Study: In Japan, AI-powered systems detected earthquakes seconds before they struck, triggering automated responses like shutting down trains and gas lines, thereby minimizing casualties and damage.
Benefits of AI and ML for Scalability and Efficiency
The integration of AI and ML in environmental monitoring offers numerous advantages:
- Automation: Automating data collection and analysis reduces human error and accelerates processes.
- Real-Time Monitoring: AI enables continuous, real-time tracking of environmental parameters.
- Cost-Effectiveness: By optimizing resources, AI lowers operational costs.
- Example: An AI system deployed in Brazil’s Amazon rainforest monitors deforestation in real-time, allowing authorities to respond swiftly to illegal logging activities.
Challenges in AI and ML Adoption in Environmental Sciences
While promising, the adoption of AI and ML in environmental sciences faces several hurdles:
- Ethical Concerns: Issues such as data privacy and bias in algorithms need to be addressed.
- Technical Limitations: Ensuring the reliability and accuracy of AI models in diverse conditions is a challenge.
- Collaboration: Successful implementation requires cooperation between technologists, scientists, and policymakers.
Future Trends in AI and Environmental Monitoring
The future of AI in environmental monitoring is bright, with emerging trends promising even greater advancements:
- Edge AI: Utilizing localized processing for faster decision-making. For instance, AI sensors in remote areas can process data without relying on cloud computing.
- Quantum Computing: Potential to solve complex environmental models at unprecedented speeds.
- IoT Integration: Combining AI with the Internet of Things (IoT) enhances data collection and analysis.
- Example: Smart cities are leveraging AI and IoT to optimize energy use, waste management, and air quality.
Conclusion:
AI and ML are transforming environmental monitoring by providing accurate, efficient, and scalable solutions to pressing ecological challenges. From wildfire detection to disaster management, these technologies are enabling proactive and informed decision-making.
By addressing challenges and fostering collaboration, AI can play a pivotal role in achieving sustainable development goals and preserving our planet for future generations. The integration of AI into environmental sciences is not just an opportunity but a necessity in the face of global environmental crises.