AI Transformations: How New Algorithms Are Redefining Industries
Artificial Intelligence (AI) has come a long way from its initial stages as a theoretical concept. Today, AI is not just an area of research but a core component of innovation across various industries. The algorithms driving AI are becoming more sophisticated, enabling machines to perform tasks that were once thought to be exclusive to humans. As these algorithms evolve, they continue to redefine industries, driving efficiencies, enhancing customer experiences, and even creating new business models.
In this article, we explore the impact of AI transformations, particularly how new algorithms are reshaping industries. We will delve into some of the latest advancements in AI algorithms and their application in real-world settings.
1. Deep Learning Algorithms: The Foundation of AI Revolution
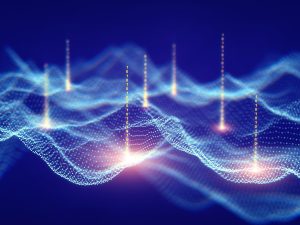
Deep learning is a subset of machine learning that has gained significant attention due to its ability to process vast amounts of data and make predictions with remarkable accuracy. These algorithms, inspired by the neural networks of the human brain, have already transformed industries such as healthcare, finance, and retail.
Benefits of Deep Learning:
- Enhanced Accuracy: Deep learning models can analyze complex patterns in data, leading to more accurate predictions and insights.
- Automation: These algorithms can automate processes that require human intelligence, such as image recognition or voice processing.
- Scalability: Deep learning models can handle enormous datasets, making them scalable for large enterprises and even global applications.
Case Study: Healthcare
One of the most significant applications of deep learning is in medical imaging. AI algorithms have been used to detect diseases like cancer, diabetes, and eye conditions with a level of accuracy comparable to, or even exceeding, that of human doctors.
For example, Google’s DeepMind developed an AI model that can detect retinal diseases from eye scans. The model can identify signs of diabetic retinopathy and age-related macular degeneration, both leading causes of blindness. The model has shown to be 94% accurate, a major breakthrough for early detection in medical practice.
Case Study: Finance
In the financial industry, deep learning is utilized to predict market trends and detect fraud. JPMorgan Chase, one of the world’s largest financial institutions, uses AI to analyze financial data and predict stock market movements. By processing historical market data, these algorithms provide predictive insights that help in making better investment decisions. Additionally, AI-driven fraud detection systems analyze transaction patterns and flag any unusual activity, minimizing the risk of fraudulent transactions.
2. Natural Language Processing (NLP): AI’s Ability to Understand and Generate Human Language
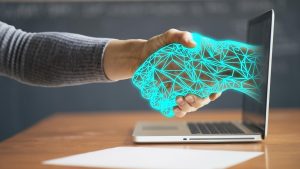
Natural Language Processing (NLP) is a crucial area of AI that focuses on enabling machines to understand and interpret human language. NLP algorithms allow computers to interact with users in a natural, conversational manner.
Benefits of NLP:
- Improved Customer Service: NLP powers chatbots and virtual assistants, enhancing customer experience through real-time, automated interactions.
- Data Insights: NLP can be used to analyze large volumes of textual data, uncovering hidden trends and patterns.
- Efficiency: Automated text generation and content creation tools can save time and improve productivity in marketing, journalism, and content creation.
Case Study: Customer Service and Chatbots
Many companies now leverage AI-powered chatbots to automate customer support, reducing the need for human agents. A prime example of NLP at work is ChatGPT, which utilizes large language models to engage in meaningful conversations with users. Businesses are using such chatbots to provide 24/7 customer support, handling common queries and resolving issues without human intervention.
For instance, Bank of America uses a virtual assistant named Erica, which helps customers with banking tasks such as paying bills, checking balances, and transferring funds. The chatbot uses NLP to understand customer queries and respond with relevant information, improving both customer satisfaction and operational efficiency.
Case Study: Content Generation in Marketing
NLP algorithms are also being used for content generation. GPT-3 from OpenAI is one of the most advanced language models and has been employed in various fields, from writing blog posts to generating product descriptions. This has helped companies automate content creation at scale.
For example, the e-commerce platform Shopify utilizes AI to generate product descriptions based on the product’s features and specifications. This allows businesses to quickly create large volumes of content for their online stores, reducing the burden on content creators and improving the overall efficiency of content production.
3. Reinforcement Learning: AI That Learns From Interaction
Reinforcement Learning (RL) is an area of machine learning where algorithms learn by interacting with their environment and receiving feedback in the form of rewards or penalties. This approach is commonly used for decision-making in real-time environments.
Benefits of Reinforcement Learning:
- Real-Time Decision Making: RL algorithms can make decisions in dynamic, unpredictable environments.
- Improved Efficiency: These algorithms can learn to optimize actions over time, leading to better long-term outcomes.
- Autonomy: RL is ideal for autonomous systems that need to operate without human intervention.
Case Study: Autonomous Vehicles
Reinforcement learning has had a transformative impact on autonomous vehicles. Companies like Tesla and Waymo are using RL to develop self-driving cars. By continually interacting with the driving environment, these cars can learn how to make safe and efficient driving decisions.
For example, Waymo, a subsidiary of Alphabet (Google’s parent company), uses RL to enable its self-driving vehicles to navigate complex urban environments. The car learns by trial and error, adjusting its actions based on feedback from sensors and cameras to avoid obstacles, obey traffic laws, and ensure passenger safety.
Case Study: Robotics in Manufacturing
Reinforcement learning is also widely used in robotics for tasks such as assembly, packaging, and even surgical procedures. For instance, Amazon Robotics uses RL to train robots to pick and pack products in its fulfillment centers. These robots learn to navigate shelves, identify products, and optimize their movements, making them increasingly efficient over time.
4. Generative Adversarial Networks (GANs): AI’s Creative Potential
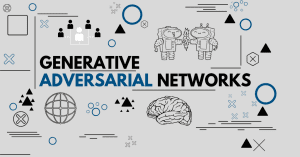
Generative Adversarial Networks (GANs) have gained attention for their ability to create new data from scratch. GANs work by using two neural networks—the generator and the discriminator—competing against each other to produce increasingly realistic outputs.
Benefits of GANs:
- Creative Content Generation: GANs can generate images, music, and even realistic faces, making them valuable in entertainment and design.
- Data Augmentation: GANs can generate synthetic data for training other AI models, reducing the need for large, labeled datasets.
- Enhancing User Experiences: GANs can help create immersive environments in video games, virtual reality, and digital arts.
Case Study: Fashion and Design
In the fashion industry, GANs are being used to generate new clothing designs and predict emerging trends. The Fabricant, a digital fashion house, uses AI to create 3D-printed garments and fashion designs that exist purely in the digital space. By leveraging GANs, they can create unique clothing items without the constraints of traditional manufacturing processes.
Case Study: Entertainment and Media
GANs have also been used in media production, particularly in generating realistic digital art and animation. For example, Disney employs GANs to create realistic human faces for animated characters in movies and TV shows. These algorithms enable artists to generate lifelike features, such as facial expressions and movements, at a fraction of the time it would take to do manually.
5. AI in Manufacturing: Predictive Maintenance and Supply Chain Optimization
AI is playing a pivotal role in transforming the manufacturing sector through predictive maintenance and supply chain optimization.
Benefits of AI in Manufacturing:
- Reduced Downtime: AI-driven predictive maintenance helps prevent machine breakdowns by predicting when maintenance is needed.
- Cost Savings: Optimizing supply chains using AI can help reduce costs associated with overstocking and understocking.
- Improved Productivity: AI enhances production line efficiency by identifying bottlenecks and optimizing workflows.
Case Study: General Electric (GE)
General Electric (GE) has incorporated AI into its manufacturing processes by using predictive maintenance algorithms to monitor the health of equipment and machinery. This proactive approach reduces unexpected downtime, allowing GE to improve its production efficiency and reduce repair costs.
Case Study: Siemens
Siemens uses AI in Industry 4.0 to optimize manufacturing processes. By integrating AI into their MindSphere platform, Siemens provides real-time data analytics to manufacturers, helping them optimize operations, predict maintenance needs, and increase production quality.
6. AI-Powered Personalization: Transforming Marketing and Customer Experience
Personalization is one of the most powerful applications of AI in marketing. AI algorithms can analyze consumer data to create personalized experiences that increase customer satisfaction and drive engagement.
Benefits of AI Personalization:
- Better Customer Engagement: Personalized recommendations and content lead to higher engagement rates.
- Increased Conversion Rates: Tailored offers and suggestions improve the likelihood of a sale.
- Enhanced Customer Loyalty: By meeting customer preferences, AI helps in building long-term relationships with clients.
Case Study: E-commerce and Retail
Amazon and Netflix are two of the most prominent examples of companies that use AI-powered personalization. Amazon uses AI to recommend products based on past purchases, browsing history, and customer reviews. Netflix, on the other hand, uses AI to suggest movies and TV shows based on users’ viewing history, increasing engagement on the platform.
Conclusion: Embracing AI for the Future
AI’s transformative potential is evident across industries, from healthcare to manufacturing, finance, and beyond. The continuous advancement of algorithms like deep learning, NLP, reinforcement learning, and GANs promises even greater innovation. For businesses, the challenge lies in adopting AI responsibly, ensuring that these technologies are used ethically while maximizing their potential for growth and efficiency.
By embracing AI, industries can unlock new possibilities, enhance customer experiences, and drive operational efficiency. The future of AI is here, and it is shaping the world around us in ways we never imagined.
FAQs
What are deep learning algorithms used for?
Deep learning algorithms are used for tasks such as medical image analysis, fraud detection, autonomous driving, and more, by analyzing vast amounts of data.
How is NLP improving customer service?
NLP enables chatbots and virtual assistants to understand and respond to customer inquiries, providing 24/7 automated support and enhancing customer satisfaction.
What is reinforcement learning used for?
Reinforcement learning is used in applications like autonomous vehicles, robotics, and dynamic decision-making systems, where the AI learns through trial and error.
How do GANs work?
GANs work by using two neural networks that generate and evaluate data, respectively. The generator creates data, and the discriminator determines if it’s real or fake, leading to the creation of high-quality outputs.
How is AI transforming the manufacturing sector?
AI is transforming manufacturing through predictive maintenance, which reduces downtime, and supply chain optimization, which improves efficiency and cost savings.