The Role of AI in Predictive Maintenance: Transforming Asset Management
In the modern industrial landscape, ensuring that machinery and equipment run efficiently is essential for minimizing downtime and maximizing productivity. Traditional maintenance strategies like reactive or scheduled maintenance often fall short, leading to unnecessary costs and potential operational disruptions. This is where predictive maintenance (PdM) powered by Artificial Intelligence (AI) comes into play, revolutionizing how companies manage their assets and ensure operational reliability.
Predictive maintenance leverages AI technologies, especially machine learning (ML), deep learning, and IoT (Internet of Things) sensors, to predict equipment failures before they occur. By doing so, it helps organizations reduce unexpected downtime, extend the lifespan of machinery, and cut maintenance costs. This article explores the role of AI in predictive maintenance, its benefits, industry-specific applications, challenges, and how organizations can harness the full potential of this technology.
Introduction to Predictive Maintenance and AI
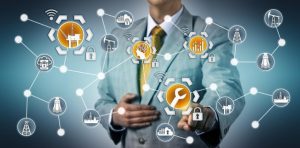
Predictive maintenance refers to using advanced data analytics and machine learning algorithms to predict when an equipment failure might occur. This allows maintenance to be performed just in time to address the issue, preventing unplanned downtime and optimizing maintenance schedules.
Traditionally, maintenance strategies were either reactive, where maintenance was performed after failure, or preventive, where maintenance was performed at set intervals regardless of the equipment’s condition. Both methods, however, have their drawbacks—reactive maintenance leads to unscheduled downtime, and preventive maintenance can result in unnecessary repairs, increasing operational costs.
AI changes this dynamic by enabling predictive maintenance, where machines communicate their status in real-time. With AI-driven predictive models, data from sensors embedded in machines is continuously analyzed to detect patterns or anomalies that may signal impending failures.
AI-Driven Condition Monitoring
AI is increasingly being integrated with IoT sensors to monitor the health of machinery in real time. These sensors collect a vast array of data, including temperature, vibration, noise, pressure, and even environmental conditions. This data is then transmitted to AI models that continuously analyze it to detect abnormal patterns indicative of potential failure.
Benefits:
- Real-Time Detection: AI allows for the detection of issues as they arise, ensuring maintenance is performed at the right moment to avoid unexpected failures.
- Early Problem Detection: Machine learning models are trained to identify even subtle anomalies that human operators might miss.
For instance, vibration data from a pump motor might reveal a gradual imbalance. AI models can detect this early, preventing a catastrophic failure by allowing maintenance crews to address the imbalance before it leads to more severe damage.
Example:
General Electric (GE) uses AI-driven condition monitoring systems in their industrial equipment, such as turbines and compressors, to predict component wear and reduce unplanned outages.
Machine Learning Algorithms for Predictive Maintenance
Machine learning (ML) algorithms play a pivotal role in predictive maintenance by analyzing historical data and identifying trends that can predict future events. These algorithms are trained on vast amounts of data gathered from equipment, enabling them to learn patterns of normal operation and flag deviations that may indicate a potential failure.
Types of Machine Learning Used:
- Supervised Learning: In this approach, ML models are trained on labeled historical data (e.g., failure events with associated causes) to predict when equipment might fail.
- Unsupervised Learning: This method helps identify unknown patterns in data that could suggest a problem, even without a prior failure history.
- Reinforcement Learning: AI models continuously adapt based on real-time feedback, improving predictive accuracy as they learn from operational data.
Benefits:
- Improved Prediction Accuracy: ML algorithms can predict failures with higher accuracy compared to traditional methods.
- Data-Driven Decisions: AI models enable data-backed decision-making for scheduling maintenance and optimizing operations.
Example:
Siemens has integrated ML-based predictive maintenance solutions into their MindSphere platform, which analyzes data from sensors across a factory to predict potential failures, thus reducing unplanned downtime by over 20%.
Deep Learning in Failure Prediction
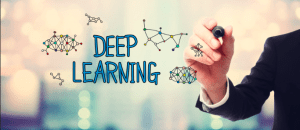
Deep learning, a subset of machine learning, uses neural networks to analyze complex data patterns. Unlike traditional ML models, deep learning can process vast amounts of unstructured data (e.g., images, sounds, and sensor readings), making it ideal for predictive maintenance.
For example, deep learning models can be trained to analyze audio recordings of machinery and identify subtle changes in sound that may indicate a developing issue, such as a worn-out bearing or a loose fan blade.
Benefits:
- Fault Classification: Deep learning can classify various types of faults based on the input data and prioritize them based on severity.
- Time-Series Analysis: Deep learning models are particularly effective at analyzing time-series data, allowing them to detect trends or irregularities that suggest an impending failure.
Case Study:
In the aerospace industry, Rolls-Royce uses deep learning algorithms to monitor the health of jet engines. By analyzing thousands of data points from sensors embedded in the engines, the system can predict the exact time when a component will need maintenance, improving aircraft reliability and reducing delays.
Digital Twin Technology and AI Integration
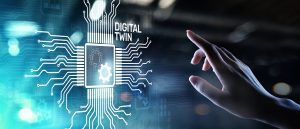
A digital twin is a virtual representation of a physical asset, such as a machine, product, or even an entire system. By integrating AI with digital twins, companies can simulate real-world behavior, predict equipment failures, and optimize maintenance schedules.
AI enhances digital twin technology by using real-time data to create accurate models of the equipment’s performance, allowing for:
- Scenario Simulation: AI-powered digital twins can simulate various scenarios (e.g., extreme conditions or heavy usage) to predict how equipment will behave under stress and when maintenance will be required.
- Predictive Maintenance Scheduling: AI enables real-time adjustments to maintenance schedules based on the digital twin’s performance predictions.
Example:
Dassault Systèmes uses AI to enhance digital twin models for manufacturing and aerospace companies. By simulating the behavior of an entire production line or individual aircraft components, organizations can schedule maintenance based on actual wear and tear, rather than arbitrary intervals.
Automating Maintenance Workflows with AI
AI doesn’t just predict failures; it also automates the entire maintenance workflow, from scheduling to resource allocation. Predictive maintenance powered by AI helps automate:
- Maintenance Scheduling: AI can automatically schedule maintenance tasks based on predictive insights, adjusting schedules dynamically as needed.
- Inventory Management: AI predicts which parts will likely be needed and ensures that spare parts are available when required.
- Workforce Management: AI optimizes technician deployment, ensuring that the right personnel are available at the right time for repairs.
Benefit:
- Efficiency Gains: Automating workflows significantly reduces human error and increases operational efficiency.
- Cost Reduction: AI-based scheduling prevents over-servicing and minimizes the cost of unnecessary repairs.
Example:
Schneider Electric, a global energy management company, has implemented AI-driven maintenance scheduling and predictive inventory management for its industrial customers, resulting in a 30% reduction in operational costs.
Cloud-Based AI Platforms for Predictive Maintenance
Cloud-based platforms provide an accessible, scalable, and cost-effective way for organizations to implement AI-driven predictive maintenance. These platforms aggregate data from multiple sources, apply AI models, and deliver actionable insights through user-friendly dashboards.
Benefits:
- Scalability: Cloud platforms enable businesses of all sizes to scale their predictive maintenance efforts without investing in expensive infrastructure.
- Real-Time Data Access: Cloud-based systems provide real-time access to data, enabling remote monitoring and diagnostics.
Example:
IBM’s Maximo is a cloud-based predictive maintenance platform that uses AI to monitor equipment, predict failures, and optimize maintenance. It has been used across industries like oil and gas, utilities, and manufacturing to reduce downtime and extend asset life.
Industry-Specific AI Applications in Predictive Maintenance
Different industries have adopted AI-based predictive maintenance in ways that suit their unique needs. Here are some key applications:
- Manufacturing: In factories, AI helps predict failures in robotics, CNC machines, and assembly lines, improving production uptime and reducing waste.
- Energy and Utilities: AI is applied in turbines, power grids, and transmission lines to predict failures and reduce service interruptions.
- Aerospace: In the aviation industry, AI predicts aircraft engine failures, reducing downtime and enhancing airworthiness.
- Automotive: AI models predict vehicle component failures, helping fleet operators optimize maintenance schedules and reduce downtime.
Case Study:
Tesla’s Gigafactory uses AI and IoT sensors to monitor the health of manufacturing robots and other critical equipment. Predictive maintenance has significantly reduced machine downtime, ensuring continuous production.
Challenges in Implementing AI for Predictive Maintenance
While AI holds great promise, several challenges exist in its implementation:
- Data Quality and Integration: Poor-quality or fragmented data can lead to inaccurate predictions. Effective data integration across systems is crucial.
- High Initial Investment: Deploying AI-based predictive maintenance solutions requires substantial upfront costs in sensors, software, and infrastructure.
- Interpretability of AI Models: Deep learning models, in particular, can be difficult to interpret, which raises concerns in industries where safety is a critical issue.
The Future of AI in Predictive Maintenance
As AI technologies continue to evolve, the future of predictive maintenance looks promising. Key trends to watch include:
- Reinforcement Learning: AI systems that continuously learn and adapt from real-time data will make predictive maintenance even more precise.
- AI-Powered Autonomous Systems: In the future, autonomous robots may perform maintenance tasks based on AI predictions, further reducing the need for human intervention.
- Integration with AR/VR: Augmented reality (AR) and virtual reality (VR) could be integrated with predictive maintenance platforms to provide real-time visual guidance to technicians during repairs.
Conclusion
AI-powered predictive maintenance is transforming how industries manage equipment and assets, offering significant benefits in terms of cost reduction, uptime optimization, and operational efficiency. By integrating machine learning, deep learning, IoT, and cloud-based platforms, organizations can predict failures before they occur, schedule maintenance effectively, and reduce unnecessary downtime. While challenges like data quality, initial investment, and model interpretability exist, the future of predictive maintenance holds immense potential for further innovations that will shape the next generation of asset management.
As industries continue to embrace these technologies, companies that invest in AI-driven predictive maintenance will be better equipped to stay ahead of the competition and achieve long-term operational success.
This article provides a thorough and comprehensive exploration of AI’s role in predictive maintenance, with real-world examples and case studies to highlight its practical applications. It is designed to be informative, accessible, and highly readable, making it suitable for both industry professionals and those looking to understand this emerging technology.