In today’s rapidly evolving financial landscape, managing risk has become more critical than ever. Traditional methods of risk management are no longer sufficient to address the complexities of modern finance. Enter Big Data—a transformative force reshaping how financial institutions identify, analyze, and mitigate risks. This article delves into the profound impact of Big Data on Financial Risk Management, offering insights into its benefits, challenges, and future prospects.
The Role of Big Data in Financial Risk Management
1. Understanding Financial Risk Management
Financial risk management involves identifying potential threats to an organization’s financial health and devising strategies to minimize these risks. These can include market risks, credit risks, operational risks, and liquidity risks. Traditionally, this relied heavily on historical data and expert judgment, but with the advent of Big Data, the scope and accuracy of risk management have expanded significantly.
2. What is Big Data?
Big Data refers to vast volumes of structured and unstructured data generated at unprecedented speeds from various sources like financial transactions, social media, and IoT devices. Big Data analytics employs advanced tools and algorithms to process, analyze, and derive insights from this data.
3. How Big Data Enhances Risk Management
Big Data provides a comprehensive view of potential risks by integrating data from multiple sources. It allows institutions to:
- Predict market fluctuations with greater accuracy.
- Detect fraudulent activities in real-time.
- Assess creditworthiness using non-traditional data sources.
- Enhance decision-making through predictive modeling and scenario analysis.
Advantages of Using Big Data in Financial Risk Management
1. Improved Predictive Analysis
Big Data enables predictive analytics, helping financial institutions foresee potential risks before they materialize. For instance, machine learning models can predict loan defaults by analyzing past behavior and external factors like market trends.
2. Real-Time Risk Detection
Unlike traditional systems that analyze data periodically, Big Data allows for real-time monitoring. This is crucial in detecting fraud, as patterns can be identified and addressed instantaneously.
3. Enhanced Decision-Making
Data-driven insights improve the accuracy and reliability of decisions. Big Data analytics provides financial managers with actionable insights, enabling them to craft more effective risk mitigation strategies.
4. Cost Efficiency
Although implementing Big Data solutions may require an initial investment, the long-term benefits include reduced operational costs, fewer losses due to fraud, and optimized resource allocation.
Challenges in Implementing Big Data
1. Data Privacy Concerns
The integration of vast data sets raises significant concerns about privacy and compliance. Financial institutions must ensure they adhere to data protection regulations like GDPR or CCPA.
2. Complexity in Data Management
Handling unstructured data from diverse sources can be challenging. Financial institutions need robust infrastructure and skilled professionals to manage and process such data effectively.
3. Cybersecurity Risks
While Big Data helps in detecting risks, it also opens avenues for cyber threats. The larger the data pool, the greater the exposure to potential breaches.
4. High Implementation Costs
Adopting Big Data analytics requires significant investment in technology, training, and infrastructure, which may not be feasible for smaller institutions.
Comparative Analysis of Traditional and Big Data-Driven Risk Management
Aspect | Traditional Risk Management | Big Data-Driven Risk Management |
---|---|---|
Data Sources | Historical data and expert judgment | Real-time data from diverse sources |
Speed of Analysis | Time-consuming | Instantaneous |
Accuracy | Limited to past trends | High due to predictive modeling |
Cost Efficiency | Relatively costlier in the long run | Cost-effective over time |
Fraud Detection | Reactive | Proactive and real-time |
Future Prospects of Big Data in Financial Risk Management
1. Artificial Intelligence and Machine Learning Integration
AI and ML technologies are expected to work hand-in-hand with Big Data to create more dynamic and adaptive risk management frameworks.
2. Blockchain for Data Security
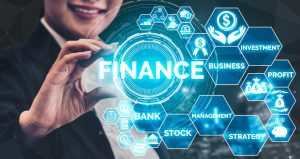
Blockchain technology can enhance the security and transparency of Big Data applications, reducing risks associated with cyber threats.
3. Greater Personalization
Big Data analytics will enable financial institutions to tailor risk management strategies to individual customer profiles, ensuring more precise and personalized solutions.
4. Regulatory Technology (RegTech)
Big Data will play a pivotal role in compliance and regulation management, enabling organizations to adhere to laws more effectively and reduce penalties.
Analysis Table
Area | Impact of Big Data | Examples |
---|---|---|
Market Risk | Improved market predictions | Stock market crash warnings |
Credit Risk | Enhanced credit assessments | Alternative data sources for loan approval |
Operational Risk | Real-time fraud detection | Identifying unusual transaction patterns |
Liquidity Risk | Accurate liquidity forecasts | Monitoring cash flow in real-time |
Leveraging Big Data for Stress Testing
Stress testing is a vital tool in financial risk management that evaluates how financial institutions would perform under extreme market conditions. Big Data enhances this process by providing a wealth of data inputs for more comprehensive scenarios. For example, using Big Data analytics, institutions can simulate global economic shocks or sector-specific downturns, ensuring preparedness for a wide range of potential crises.
Big Data in Fraud Detection
Fraudulent activities pose a significant risk to financial institutions, and Big Data is proving invaluable in combating this threat. Advanced algorithms can analyze vast amounts of transaction data to identify irregular patterns and flag suspicious activities in real-time. For instance, sudden large withdrawals or transactions in unusual locations can trigger alerts, enabling swift intervention to minimize losses.
The Role of Big Data in Portfolio Risk Management
Big Data also plays a crucial role in portfolio management by analyzing asset performance and market trends. Investors can use Big Data insights to diversify their portfolios, reduce exposure to high-risk assets, and maximize returns. Additionally, it helps in assessing the risk-return trade-off more accurately, aiding in informed decision-making.
Big Data and Compliance Management
Regulatory compliance is a critical aspect of financial risk management. Big Data simplifies this by automating the process of monitoring transactions and reporting suspicious activities. Financial institutions can use data analytics tools to ensure adherence to regulations, avoiding hefty fines and reputational damage. Furthermore, it facilitates transparent reporting, strengthening trust with stakeholders and regulators.
Big Data’s Impact on Customer Risk Profiling
Incorporating Big Data allows financial institutions to develop detailed customer risk profiles. By analyzing transaction history, spending habits, and external data sources like social media activity, institutions can gain deeper insights into a customer’s financial behavior. This helps in tailoring risk management strategies and offering personalized financial products that align with individual risk appetites.
Challenges of Data Integration in Risk Management
Integrating diverse data sources into a unified framework poses significant challenges for financial institutions. Structured and unstructured data from internal and external sources must be harmonized to derive actionable insights. Ensuring data quality, consistency, and accuracy is vital, as errors in data processing can lead to flawed risk assessments. Overcoming these challenges requires robust data management systems and skilled professionals.
Real-Time Market Sentiment Analysis with Big Data
One of the innovative applications of Big Data in financial risk management is the analysis of market sentiment. By monitoring social media trends, news outlets, and public forums, financial institutions can gauge investor sentiment in real time. This insight enables them to anticipate market movements and respond proactively to potential risks, such as sudden sell-offs or price volatility in specific sectors.
Big Data and Credit Scoring Innovations
Big Data is transforming traditional credit scoring systems by incorporating alternative data sources. These include utility payments, rental histories, and even social media activity. By leveraging this diverse data, financial institutions can evaluate creditworthiness more accurately, making loans accessible to a broader audience while minimizing the risk of defaults.
Predictive Maintenance in Operational Risk Management
Big Data is not just about financial metrics—it also contributes to operational efficiency. For example, predictive maintenance uses data from operational processes to forecast equipment failures or system downtimes. This reduces operational risks, as institutions can address potential issues before they disrupt services, ensuring smoother operations and cost savings.
Enhancing Decision-Making Through Data Visualization
Data visualization tools powered by Big Data make it easier for decision-makers to interpret complex risk assessments. Dashboards and visual analytics present critical data insights in user-friendly formats, allowing stakeholders to quickly understand potential risks and formulate strategies. This ensures that decisions are based on clear, actionable information rather than overwhelming data sets.
The Role of Big Data in Cyber Risk Management
As cyber threats become more sophisticated, Big Data is proving essential in identifying vulnerabilities within financial systems. Advanced analytics can detect unusual network activity or unauthorized access attempts, enabling institutions to mitigate cyber risks before they escalate. This proactive approach safeguards sensitive data and protects financial assets from digital threats.
Big Data in Forecasting Global Economic Trends
On a macroeconomic level, Big Data is helping financial institutions understand and forecast global economic trends. By analyzing trade data, geopolitical events, and international market movements, institutions can prepare for economic disruptions. This capability is especially valuable for multinational organizations managing risks across diverse markets and currencies.
Conclusion
Big Data is undeniably revolutionizing financial risk management, making it more efficient, precise, and proactive. While challenges exist, the potential benefits far outweigh the hurdles. By leveraging Big Data, financial institutions can safeguard themselves against uncertainties and make more informed decisions, ensuring long-term stability and success.