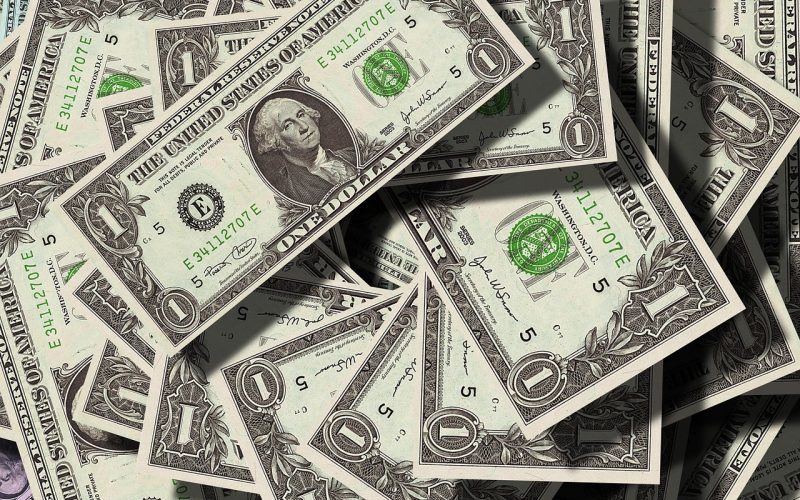
In recent years, the term “Big Data” has become a buzzword in various Financial Decision industries, including finance. Big Data refers to the vast amount of structured and unstructured data generated every day. The rise of advanced analytics and machine learning techniques has allowed financial institutions to harness this data for making smarter, faster, and more accurate decisions. This article explores the role of Big Data in financial decision-making, analyzing how it has revolutionized the finance industry and what the future holds for Big Data in finance.
Understanding Big Data in Finance
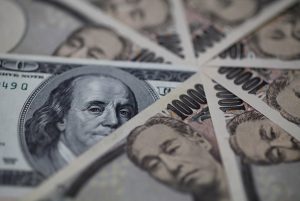
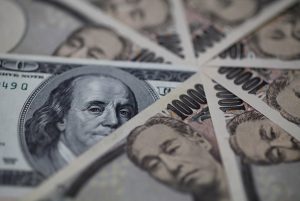
Big Data is defined by three main characteristics: volume, velocity, and variety. Financial institutions deal with enormous datasets that are generated in real time from different sources, such as market transactions, social media activity, mobile payments, and customer interactions. These data sets are too complex to be processed by traditional data management tools.
In the context of finance, Big Data is more than just vast quantities of information; it’s the ability to extract meaningful insights from this data that impacts decision-making. Financial institutions can gain a competitive edge by analyzing customer behavior, market trends, and economic indicators in real time, allowing for better forecasting, risk management, and personalized financial services.
The Impact of Big Data on Financial Decision-Making
1. Enhancing Risk Management
Risk management is one of the most critical aspects of financial decision-making. Big Data enables financial institutions to analyze vast amounts of historical data to predict and mitigate potential risks. Traditional risk management relied heavily on retrospective data, but Big Data allows real-time monitoring and predictive analytics, enabling institutions to take preemptive measures.
By analyzing data from multiple sources, such as market movements, customer behavior, and economic indicators, financial firms can identify patterns and anomalies that could indicate potential risks, such as loan defaults or fraudulent activities. This level of risk assessment helps financial institutions protect their assets and safeguard their clients’ investments.
2. Improving Investment Strategies
Big Data has transformed how investment strategies are developed. Investors and financial advisors no longer rely solely on past performance or basic market indicators. By analyzing real-time data from social media, news platforms, and global markets, Big Data helps identify market trends, forecast stock prices, and develop more dynamic investment portfolios.
For instance, hedge funds and asset managers use Big Data to perform sentiment analysis on social media to understand how public opinion may impact stock performance. Predictive analytics models also help detect hidden opportunities, allowing investors to make more informed decisions.
3. Streamlining Fraud Detection and Prevention
Fraudulent activities have always been a significant concern for the financial industry. Big Data enables the detection and prevention of fraud through real-time analytics. By analyzing transaction data, patterns, and anomalies, financial institutions can quickly identify suspicious activities and prevent fraudulent transactions before they occur.
For example, if a bank detects an unusual spending pattern on a customer’s credit card, Big Data algorithms can flag it for review and take immediate action to prevent further fraudulent activity. This proactive approach has drastically reduced the time and cost associated with fraud detection.
The Role of Predictive Analytics in Financial Forecasting
One of the most significant advantages Big Data brings to financial decision-making is the ability to leverage predictive analytics. Predictive analytics refers to using statistical models and machine learning algorithms to forecast future events based on historical data.
1. Market Forecasting
Big Data’s ability to predict market trends is invaluable for financial institutions. By analyzing vast datasets, such as consumer sentiment, economic indicators, and historical stock prices, predictive analytics can forecast market movements and guide investors on the best course of action.
2. Customer Behavior Forecasting
Financial institutions can use predictive analytics to forecast customer behaviors, such as loan repayment likelihood, spending habits, or risk of churn. By understanding customer behavior patterns, banks and financial institutions can offer personalized services, improving customer satisfaction and retention.
3. Credit Scoring
Traditional credit scoring models relied on limited data, such as an individual’s credit history. With Big Data, credit scoring has become more sophisticated, incorporating various data points like income levels, spending habits, and even social media behavior. This allows lenders to make more accurate credit assessments and reduce the risk of bad loans.
Challenges of Implementing Big Data in Finance
1. Data Privacy and Security Concerns
One of the main challenges in implementing Big Data in finance is ensuring data privacy and security. Financial institutions collect a vast amount of sensitive customer data, and protecting this data from breaches or misuse is critical. Data encryption, regulatory compliance, and secure data storage are all necessary to mitigate these risks.
2. Integration with Legacy Systems
Many financial institutions still rely on outdated legacy systems that are not designed to handle Big Data. Integrating modern Big Data analytics platforms with these systems can be challenging and costly. Financial firms must invest in updating their IT infrastructure to fully harness the benefits of Big Data.
3. Data Quality and Accuracy
Another challenge is ensuring the quality and accuracy of the data being analyzed. Big Data is often unstructured and comes from various sources, making it difficult to ensure that the data is accurate and relevant. Financial institutions must invest in data cleaning and validation processes to ensure the quality of their analytics.
Future Trends in Big Data Finance
1. Artificial Intelligence and Machine Learning Integration
As artificial intelligence (AI) and machine learning (ML) technologies continue to evolve, their integration with Big Data in finance will further enhance financial decision-making. These technologies can automate complex analyses and improve the accuracy of predictions, allowing financial institutions to respond more quickly to market changes and customer needs.
2. Blockchain and Big Data
The combination of blockchain technology and Big Data could revolutionize the financial industry. Blockchain’s decentralized and transparent nature makes it an excellent partner for Big Data analytics, ensuring data integrity and improving fraud prevention.
3. Real-Time Data Processing
The future of Big Data finance lies in real-time data processing. As more financial services go digital, the ability to analyze data in real time will be critical for maintaining a competitive edge. Financial institutions that can analyze market trends, customer behavior, and economic data in real time will be able to make faster and more informed decisions.
Analysis Table: Key Roles of Big Data in Financial Decision-Making
Role of Big Data | Explanation |
---|---|
Risk Management | Predicts risks and mitigates potential financial losses through real-time data analysis. |
Investment Strategies | Helps investors forecast stock prices and identify hidden market opportunities. |
Fraud Detection | Detects and prevents fraudulent activities by analyzing transaction patterns. |
Predictive Analytics | Forecasts future market trends, customer behaviors, and credit risk. |
Credit Scoring | Enhances credit scoring by incorporating non-traditional data points. |
Comparative Table: Traditional vs. Big Data Approaches in Finance
Aspect | Traditional Finance Approach | Big Data Finance Approach |
---|---|---|
Data Analysis | Limited to historical and structured data | Utilizes both structured and unstructured data in real time |
Risk Management | Based on retrospective data analysis | Predictive risk assessment with real-time monitoring |
Investment Strategies | Relies on past performance and basic indicators | Analyzes real-time global data, including social sentiment and news feeds |
Fraud Detection | Manual detection and delayed response | Automated, real-time fraud detection |
Credit Scoring | Based on limited credit history and financial data | Uses a variety of data points like spending habits and social behavior |
Conclusion
Big Data has already proven to be a game-changer in the financial industry, enabling better risk management, improved investment strategies, and enhanced fraud detection. As financial institutions continue to adopt and refine Big Data technologies, the impact on financial decision-making will only grow. However, challenges such as data privacy, system integration, and data quality need to be addressed to fully realize the potential of Big Data in finance.
The future holds exciting possibilities for Big Data in finance, particularly as AI, machine learning, and blockchain technologies continue to develop. By staying ahead of these trends and investing in robust Big Data strategies, financial institutions can ensure they remain competitive in an ever-evolving landscape.