Transforming Transformers: A Leap in Language Model Efficiency
Introduction
Welcome to an exploration of the latest advancements in Transformer models. This article is penned by Fred Wilson, an AI researcher with over a decade of experience in Machine Learning and Natural Language Processing. His expertise lies in optimizing language models, and he’s here to share his insights on the transformative advancements in language model efficiency.
The Evolution of Language Models
Language models have made significant strides over the past two decades, evolving in tandem with advancements in AI. From Recurrent Neural Networks (RNNs) to Transformers, each iteration has brought us closer to achieving real-time translation, a long-standing goal for linguists. Today, tools like Google Translate, which supports 109 languages in text-to-text translation, serve as communication bridges across the globe.
Understanding Transformers
Transformers, unlike RNNs, process data all at once rather than in sequence. This approach has not only increased processing speed but also reduced the amount of processing power used. Transformers have proven so useful in Natural Language Processing (NLP) that researchers are now extending their application to other types of input, like visual inputs.
The Efficiency Challenge in Transformers
Despite their advantages, Transformers face challenges in terms of computational and memory efficiency. These challenges stem from the fact that Transformers, being a flexible architecture, make few assumptions about the structural bias of input data.
Recent Breakthroughs in Transformer Efficiency
The broader application of Transformers, such as GPT-3 for NLP and its evolution GLaM, Gopher, MT-NLG, ViT for image recognition, is expected to accelerate as more data spaces are processed and become available. This expansion is also supported by the evolution of hardware chips, like those recently announced by NVIDIA.
Case Study: GPT-3 vs GPT-Neo
GPT-3, or Generative Pre-trained Transformer 3, is a prime example of a Transformer. It can understand a summary of an article you plan to write and generate the full article, accessing knowledge available on the web. GPT-3 can even take cues from the way you express ideas in the summary and work out an article that reinforces those statements.
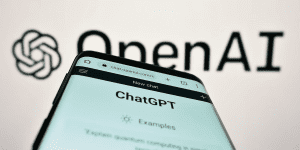
Future Directions for Efficient Transformers
The future of Transformers looks promising, with researchers continually developing and applying them in various new applications. In a paper published in August 2021, researchers from Stanford identified Transformers as “foundation models” because they believe it will transform artificial intelligence.
Conclusion: The Impact of Efficient Transformers
The impact of efficient Transformers is far-reaching. They have the potential to revolutionize fields ranging from natural language processing to image recognition. As we continue to push the boundaries of what’s possible with Transformers, we can look forward to a future where AI is more efficient, effective, and accessible than ever before.
Table for Key Points:
Key Point | Description |
---|---|
Evolution of Language Models | Tracing the journey from RNNs to Transformers |
Understanding Transformers | Deep dive into the architecture and working of Transformers |
Efficiency Challenge | Discussing the computational and memory challenges in Transformers |
Recent Breakthroughs | Highlighting the latest advancements in improving Transformer efficiency |
Case Study | Comparing the efficiency of GPT-3 and GPT-Neo |
Future Directions | Exploring potential areas of research in Transformer efficiency |